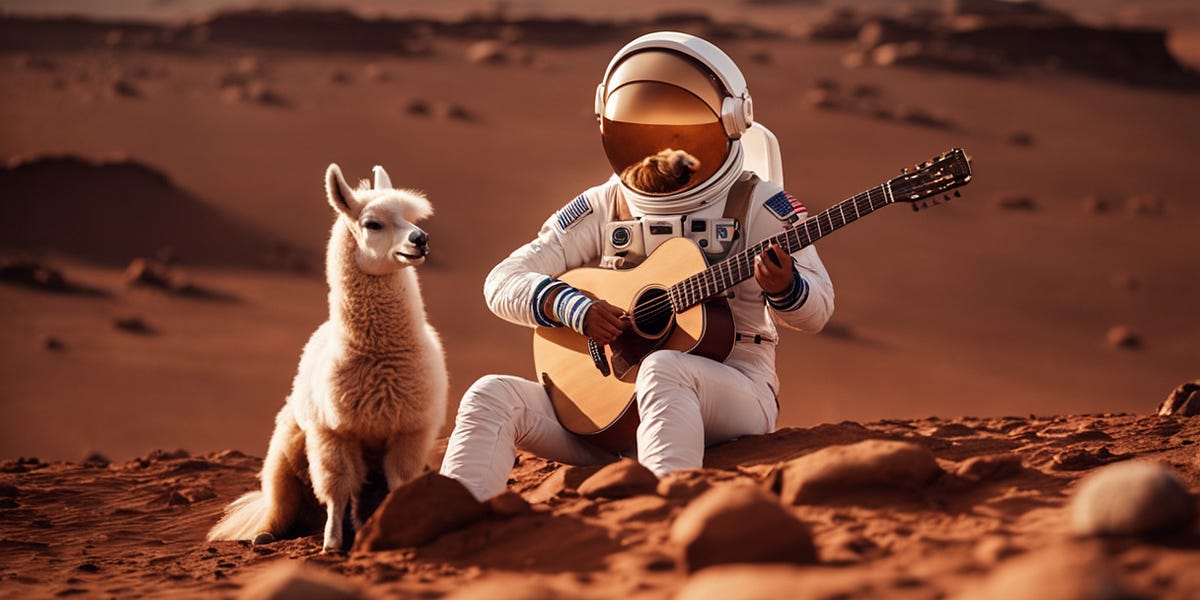
Why Open Source AI Will Win
You shouldn't bet against the bazaar or the GPU p̶o̶o̶r̶ hungry.

Why Open Source AI Will Win
You shouldn't bet against the bazaar or the GPU p̶o̶o̶r̶ hungry.
VARUN
SEP 15, 2023
Linux is subversive. Who would have thought even five years ago (1991) that a world-class operating system could coalesce as if by magic out of part-time hacking by several thousand developers scattered all over the planet, connected only by the tenuous strands of the Internet?
Certainly not I.
opening remarks in The Cathedral and the Bazaar by Eric Raymond.
There’s a popular floating theory on the Internet that a combination of the existing foundation model companies will be the end game for AI.
In the near future, every company will rent a “brain” from a model provider, such as OpenAI/Anthropic, and build applications that build on top of its cognitive capabilities.
In other words, AI is shaping up to be an oligopoly of sorts, with only a small set of serious large language model (LLM) providers.
I don’t think this could be farther from the truth. I truly believe that open source will have more of an impact on the future of LLMs and image models than the broad public believes.
There are a few arguments against open source that I see time and time again.
- Open source AI cannot compete with the resources at industry labs. Building foundation models is expensive, and non-AI companies looking to build AI features will outsource their intelligence layer to a company that specializes in it. Your average company cannot scale LLMs or produce novel results the same way a well capitalized team of talented researchers can. On the image generation side, Midjourney is miles ahead of anything else.
- Open source AI is not safe. Mad scientists cooking up intelligence on their cinderblock-encased GPUs will not align their models with general human interests
1
.
- Open source AI is incapable of reasoning. Not only do open source models perform more poorly than closed models on benchmarks, but they also lack emergent capabilities, those that would enable agentic workflows, for example.
While they seem reasonable, I think these arguments hold very little water.
LLMs are business critical
Outsourcing a task is fine — when the task is not business critical.Infrastructure products save users from wasting money and energy on learning Kubernetes or hiring a team of DevOps engineers. No company should have to hand-roll their own HR/bill payments software. There are categories of products that enable companies to “focus on what makes their beer taste better”
2
.
LLMs, for the most part, do not belong in this category. There are some incumbents building AI features on existing products, where querying OpenAI saves them on hiring ML engineers. For them, leveraging closed AI makes sense.
However, there’s a whole new category of AI native businesses for whom this risk is too great. Do you really want to outsource your core business, one that relies on confidential data, to OpenAI or Anthropic? Do you want to spend the next few years of your life working on a “GPT wrapper”?
Obviously not.
If you’re building an AI native product, your primary goal is getting off of OpenAI as soon as you possibly can. Ideally, you can bootstrap your intelligence layer using a closed source provider, build a data flywheel from engaged users, and then fine-tune your own models to perform your tasks with higher accuracy, less latency, and more control.
Every business needs to own their core product, and for AI native startups, their core product is a model trained on proprietary data
3
. Using closed source model providers for the long haul exposes an AI native company to undue risk.
There is too much pressure pent up for open source LLMs to flop. The lives of many companies are at stake. Even Google has acknowledged that they have no moat in this new world of open source AI.
Reasoning doesn’t actually matter
The general capabilities of LLMs open them up to an exponential distribution of use cases. The most important tasks are fairly straightforward: summarization, explain like I’m 5, create a list (or some other structure) from a blob of text, etc.Reasoning, the type you get from scaling these models to get larger, doesn’t matter for 85% of use cases. Researchers love sharing that their 200B param model can solve challenging math problems or build a website from a napkin sketch, but I don’t think most users (or developers) have a burning need for these capabilities.
The truth is that open source models are incredibly good at the most valuable tasks, and can be fine-tuned to cover likely up to 99% of use-cases when a product has collected enough labeled data.
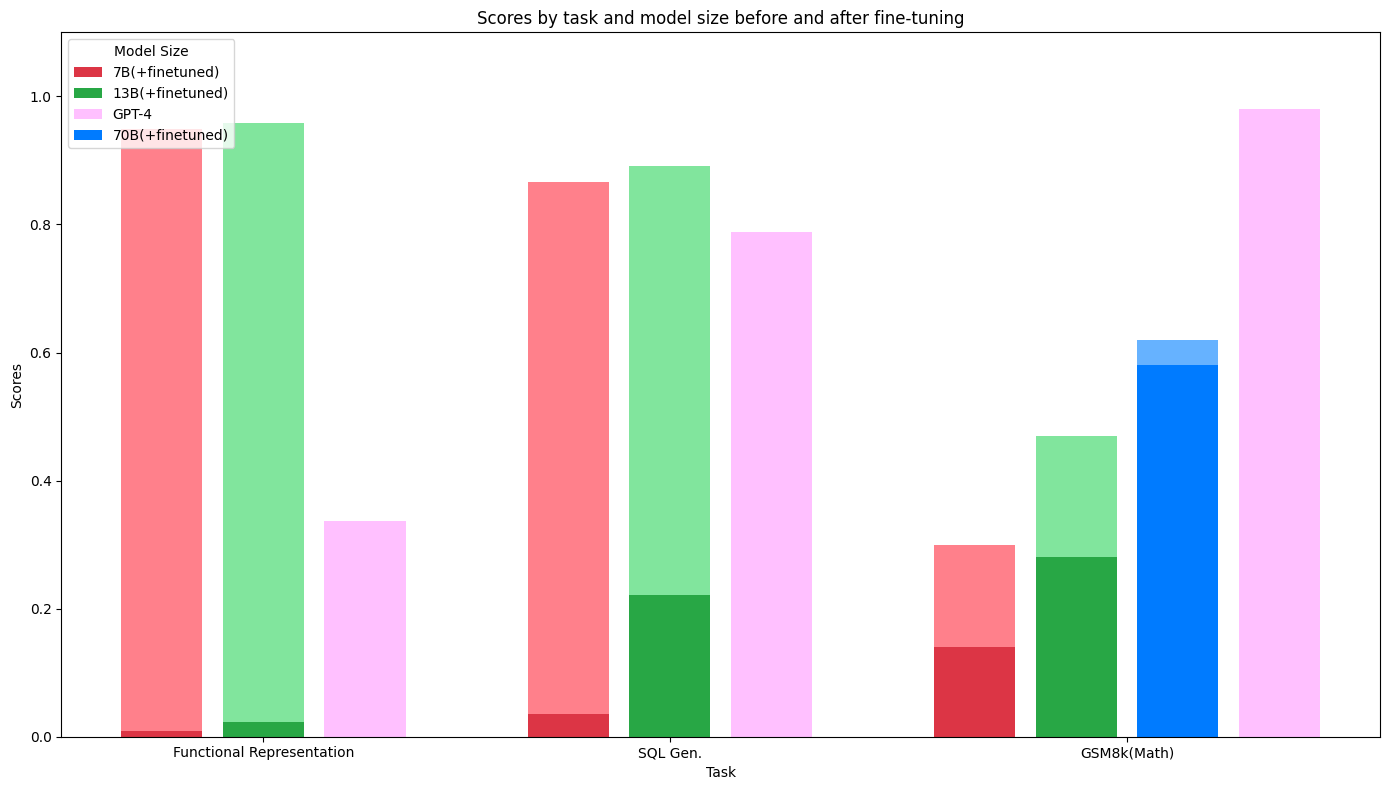
Fine-tuned Llama 2 models vs. GPT-4 (from Anyscale)
Reasoning, the holy grail that researchers are chasing, probably doesn’t matter nearly as much as people think.
More important than reasoning is context length and truthfulness.
Let’s start with context length. The longer the context length for a language model, the longer the prompts and chat logs you can pass in.
The original Llama has a context length of 2k tokens. Llama 2 has a context length of 4k.
Earlier this year, an indie AI hacker discovered that a single line code change to the RoPE embeddings for Llama 2 would give you up to 8K of context length for free with no additional training.
Just last week another indie research project was released, YaRN, that extends Llama 2’s context length to 128k tokens.
I still don’t have access to GPT-4 32k. This is the speed of open source.
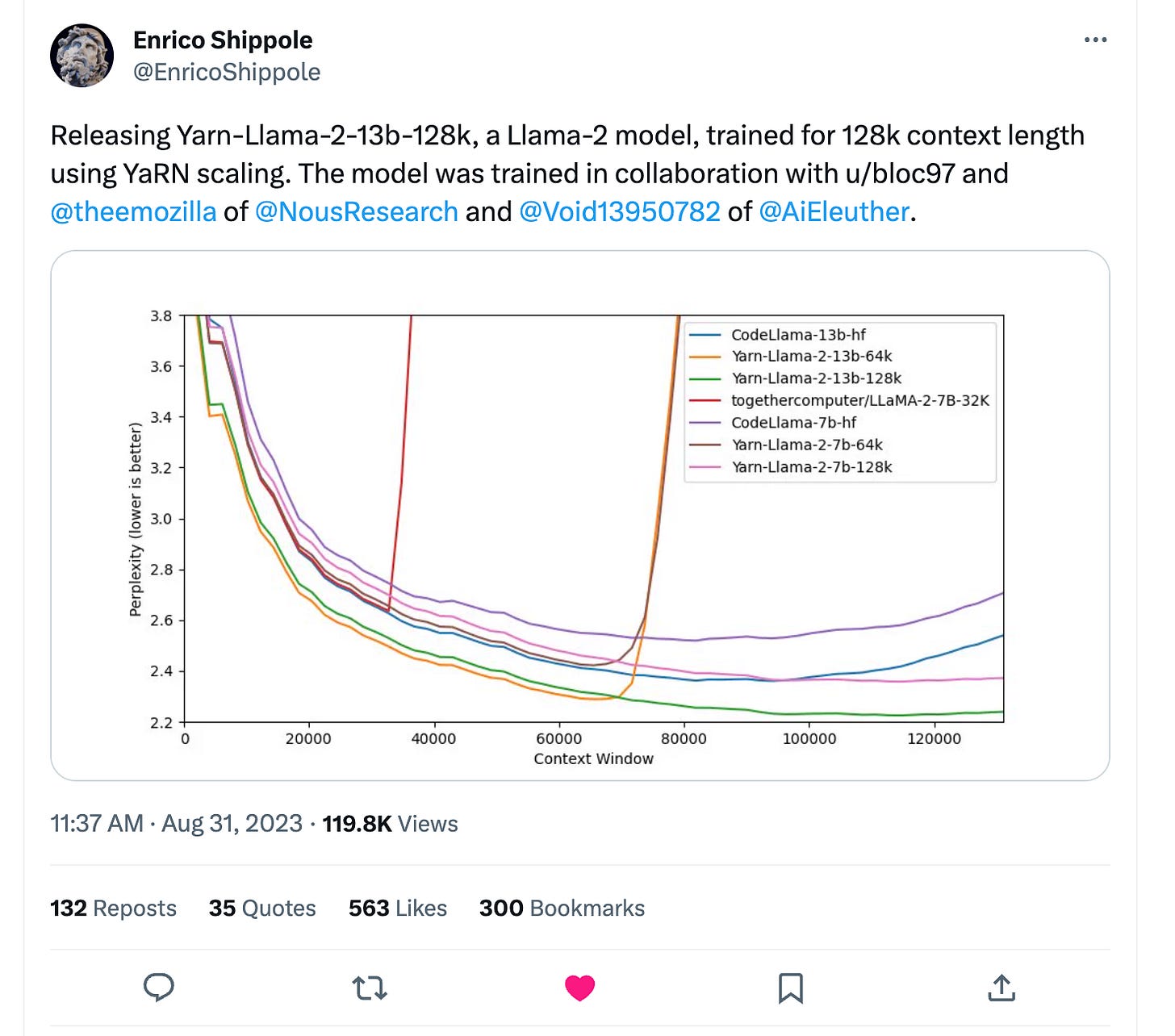
While contexts have scaled up, the hardware requirements to run massive models have also scaled down. You can now run state-of-the-art massive language models from your Macbook thanks to projects like Llama.cpp. Being able to use these models locally is a huge plus for security and costs as well. In the limit, you can run your models on your users’ hardware. Models are continuing to scale down while retaining quality. Microsoft’s Phi-1.5 is only 1.3 billion parameters but meets Llama 2 7B on several benchmarks. Open source LLM experimentation will continue to explode as consumer hardware and the GPU poor rise to the challenge.
On truthfulness: out-of-the-box open source models are less truthful than closed source models, and I think this is actually fine. In many cases, hallucination can be a feature, not a bug, particularly when it comes to creative tasks like storytelling.
Closed AI models have a certain filter that make them sound artificial and less interesting. MythoMax-L2 tells significantly better stories than Claude 2 or ChatGPT, at only 13B parameters. When it comes to honestly, the latest open source LLMs work well with retrieval augmented generation, and they will only get better.
Control above all else
Let’s take a brief look at the image generation side.I would argue that Stable Diffusion XL (SDXL), the best open source model, is nearly on-par with Midjourney.
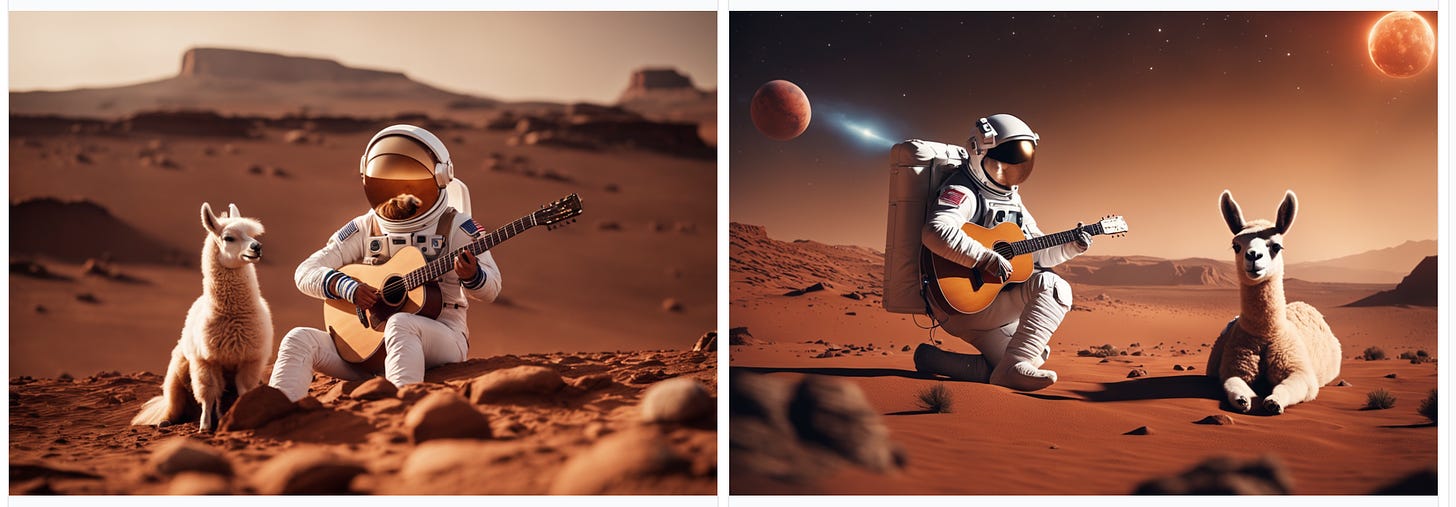
Stable Diffusion XL generations for the prompt “an astronaut playing a guitar on Mars with a llama”. These images were generated on the first try, no cherry-picking needed.
In exchange for the slightly worse ergonomics, Stable Diffusion users have access to hundreds of community crafted LoRAs
4
, fine-tunes, and textual embeddings. Users quickly discovered hands were a sore for SDXL, and within weeks a LoRA that fixes hands appeared online.
Other open source projects like ControlNet give Stable Diffusion users significantly more power when it comes to structuring their outputs, where Midjourney falls flat.
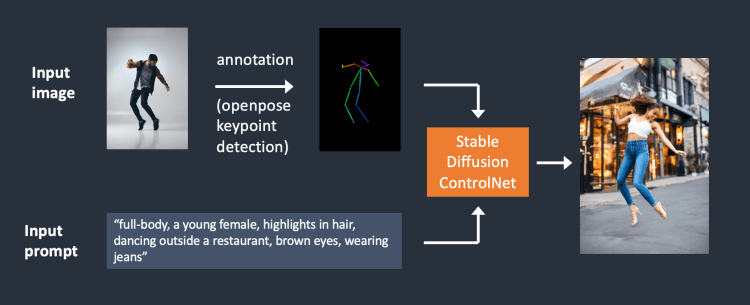
A flowchart of how Stable Diffusion + ControlNet works. Clipped from here.
Moreover, Midjourney doesn’t have an API, so if you want to build a product with an image diffusion feature, you would have to use Stable Diffusion in some form.
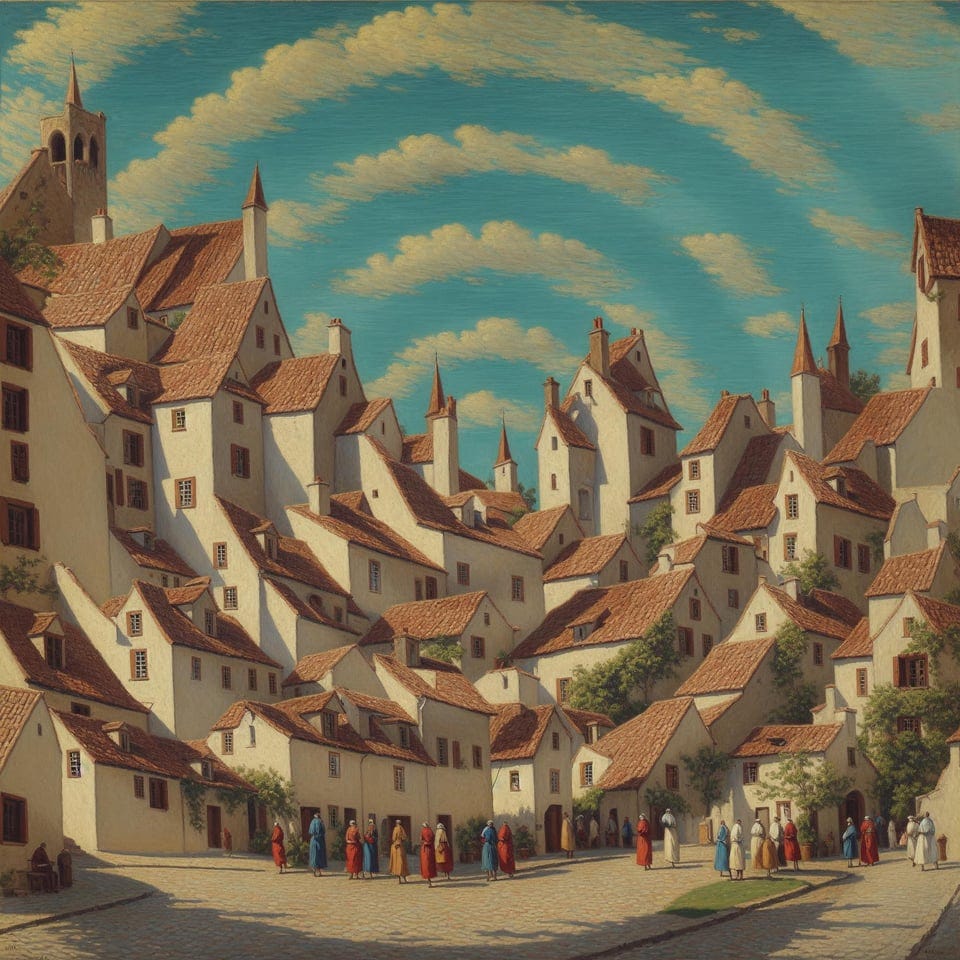
This image went viral on Twitter and Reddit this week. It uses Stable Diffusion with ControlNet. Currently, you can’t create images like this on Midjourney.