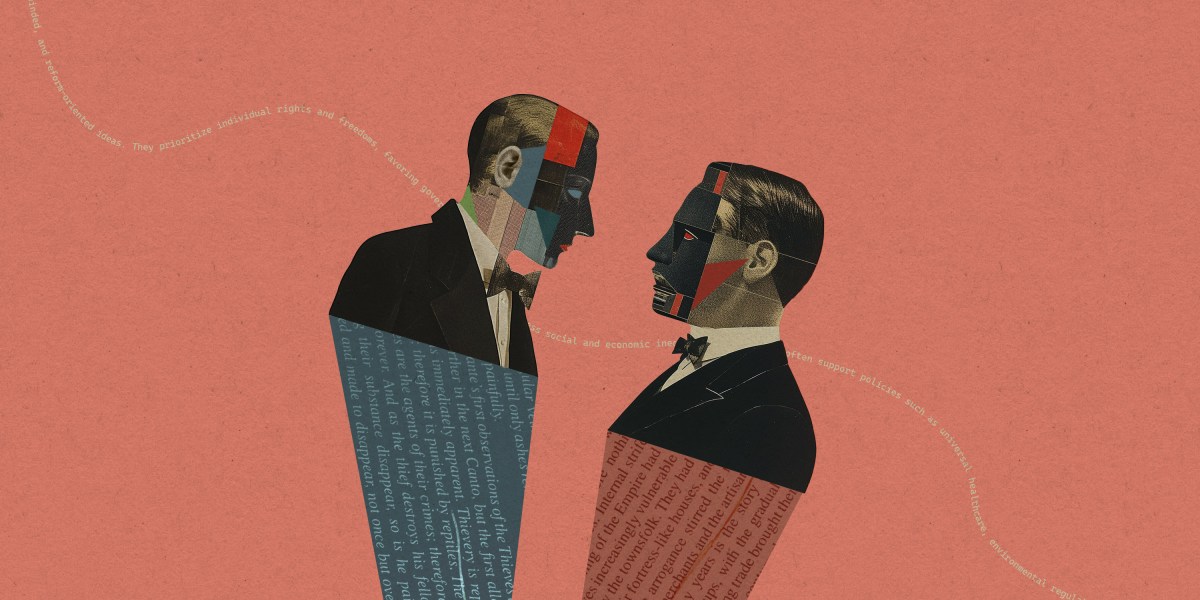
Why it’s impossible to build an unbiased AI language model
Plus: Worldcoin just officially launched. Why is it already being investigated?
ARTIFICIAL INTELLIGENCE
Why it’s impossible to build an unbiased AI language model
Plus: Worldcoin just officially launched. Why is it already being investigated?By
August 8, 2023
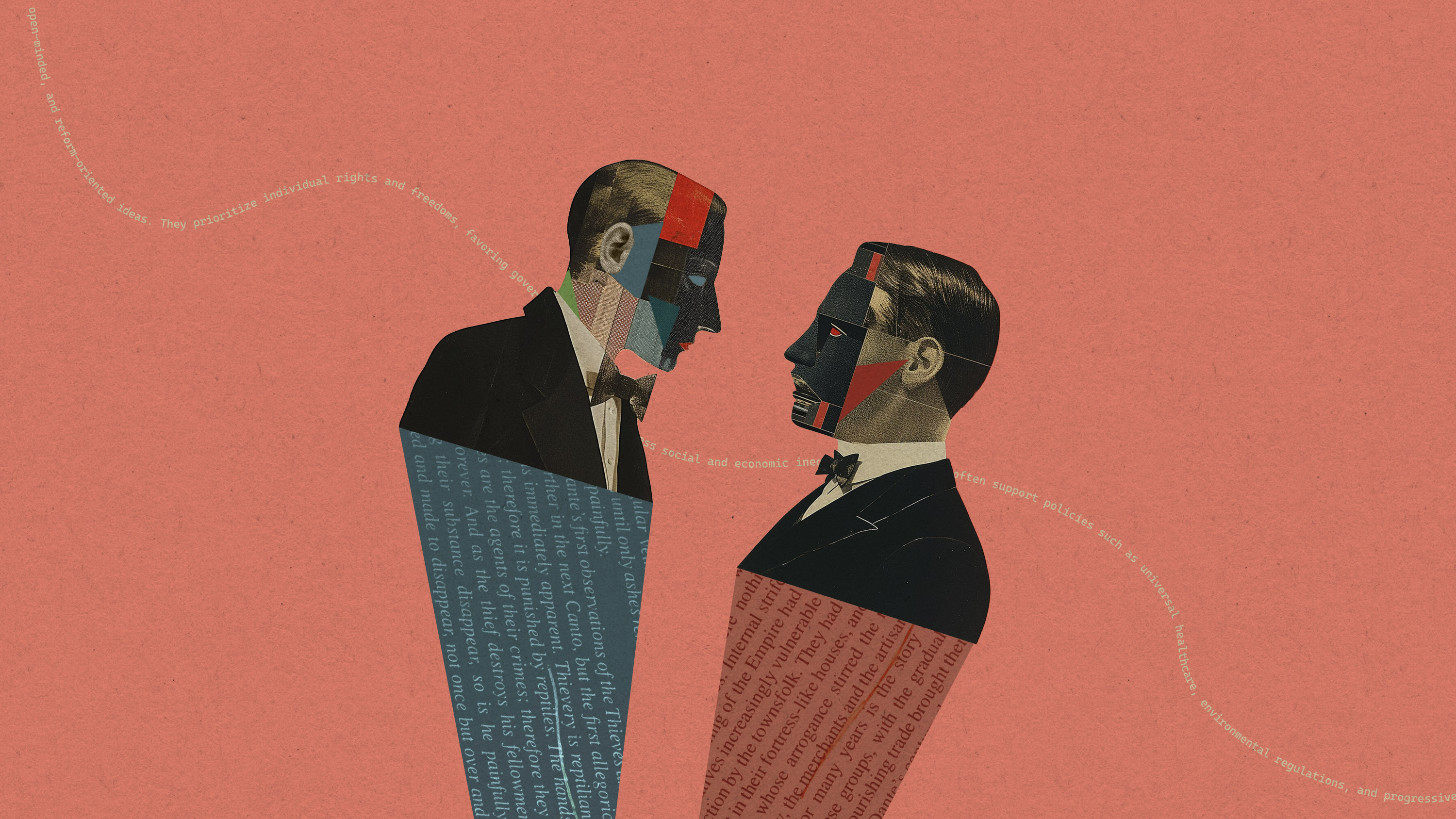
STEPHANIE ARNETT/MITTR | MIDJOURNEY (SUITS)
AI language models have recently become the latest frontier in the US culture wars. Right-wing commentators have accused ChatGPT of having a “woke bias,” and conservative groups have started developing their own versions of AI chatbots. Meanwhile, Elon Musk has said he is working on “TruthGPT,” a “maximum truth-seeking” language model that would stand in contrast to the “politically correct” chatbots created by OpenAI and Google.
An unbiased, purely fact-based AI chatbot is a cute idea, but it’s technically impossible. (Musk has yet to share any details of what his TruthGPT would entail, probably because he is too busy thinking about X and cage fights with Mark Zuckerberg.) To understand why, it’s worth reading a story I just published on new research that sheds light on how political bias creeps into AI language systems. Researchers conducted tests on 14 large language models and found that OpenAI’s ChatGPT and GPT-4 were the most left-wing libertarian, while Meta’s LLaMA was the most right-wing authoritarian.
“We believe no language model can be entirely free from political biases,” Chan Park, a PhD researcher at Carnegie Mellon University, who was part of the study, told me. Read more here.
One of the most pervasive myths around AI is that the technology is neutral and unbiased. This is a dangerous narrative to push, and it will only exacerbate the problem of humans’ tendency to trust computers, even when the computers are wrong. In fact, AI language models reflect not only the biases in their training data, but also the biases of people who created them and trained them.
And while it is well known that the data that goes into training AI models is a huge source of these biases, the research I wrote about shows how bias creeps in at virtually every stage of model development, says Soroush Vosoughi, an assistant professor of computer science at Dartmouth College, who was not part of the study.
Bias in AI language models is a particularly hard problem to fix, because we don’t really understand how they generate the things they do, and our processes for mitigating bias are not perfect. That in turn is partly because biases are complicated social problems with no easy technical fix.
That’s why I’m a firm believer in honesty as the best policy. Research like this could encourage companies to track and chart the political biases in their models and be more forthright with their customers. They could, for example, explicitly state the known biases so users can take the models’ outputs with a grain of salt.
In that vein, earlier this year OpenAI told me it is developing customized chatbots that are able to represent different politics and worldviews. One approach would be allowing people to personalize their AI chatbots. This is something Vosoughi’s research has focused on.
As described in a peer-reviewed paper, Vosoughi and his colleagues created a method similar to a YouTube recommendation algorithm, but for generative models. They use reinforcement learning to guide an AI language model’s outputs so as to generate certain political ideologies or remove hate speech.
OpenAI uses a technique called reinforcement learning through human feedback to fine-tune its AI models before they are launched. Vosoughi’s method uses reinforcement learning to improve the model’s generated content after it has been released, too.
But in an increasingly polarized world, this level of customization can lead to both good and bad outcomes. While it could be used to weed out unpleasantness or misinformation from an AI model, it could also be used to generate more misinformation.
“It’s a double-edged sword,” Vosoughi admits.
Deeper Learning
Worldcoin just officially launched. Why is it already being investigated?
OpenAI CEO Sam Altman’s new venture, Worldcoin, aims to create a global identity system called “World ID” that relies on individuals’ unique biometric data to prove that they are humans. It officially launched last week in more than 20 countries. It’s already being investigated in several of them.
Privacy nightmare: To understand why, it’s worth reading an MIT Technology Review investigation from last year, which found that Worldcoin was collecting sensitive biometric data from vulnerable people in exchange for cash. What’s more, the company was using test users’ sensitive, though anonymized, data to train artificial intelligence models, without their knowledge.
In this week’s issue of The Technocrat, our weekly newsletter on tech policy, Tate Ryan-Mosley and our investigative reporter Eileen Guo look at what has changed since last year’s investigation, and how we make sense of the latest news. Read more here.
Bits and Bytes
This is the first known case of a woman being wrongfully arrested after a facial recognition match
Last February, Porcha Woodruff, who was eight months pregnant, was arrested over alleged robbery and carjacking and held in custody for 11 hours, only for her case to be dismissed a month later. She is the sixth person to report that she has been falsely accused of a crime because of a facial recognition match. All of the six people have been Black, and Woodruff is the first woman to report this happening to her. (The New York Times)
What can you do when an AI system lies about you?
Last summer, I wrote a story about how our personal data is being scraped into vast data sets to train AI language models. This is not only a privacy nightmare; it could lead to reputational harm. When reporting the story, a researcher and I discovered that Meta’s experimental BlenderBot chatbot had called a prominent Dutch politician, Marietje Schaake, a terrorist. And, as this piece explains, at the moment there is little protection or recourse when AI chatbots spew and spread lies about you. (The New York Times)
Every startup is an AI company now. Are we in a bubble?
Following the release of ChatGPT, AI hype this year has been INTENSE. Every tech bro and his uncle seems to have founded an AI startup, it seems. But nine months after the chatbot launched, it’s still unclear how these startups and AI technology will make money, and there are reports that consumers are starting to lose interest. (The Washington Post)
Meta is creating chatbots with personas to try to retain users
Honestly, this sounds more annoying than anything else. Meta is reportedly getting ready to launch AI-powered chatbots with different personalities as soon as next month in an attempt to boost engagement and collect more data on people using its platforms. Users will be able to chat with Abraham Lincoln, or ask for travel advice from AI chatbots that write like a surfer. But it raises tricky ethical questions—how will Meta prevent its chatbots from manipulating people’s behavior and potentially making up something harmful, and how will it treat the user data it collects? (The Financial Times)