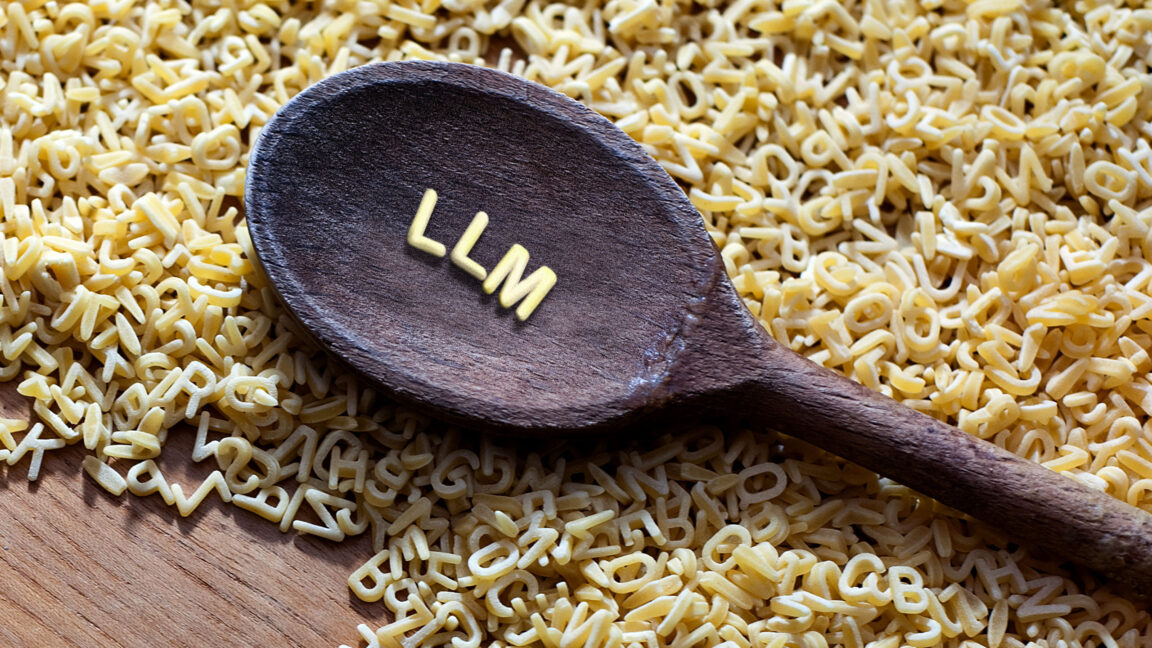
Why AI language models choke on too much text
Compute costs scale with the square of the input size. That’s not great.

Why AI language models choke on too much text
Compute costs scale with the square of the input size. That's not great.
Timothy B. Lee – Dec 20, 2024 8:00 AM | 39
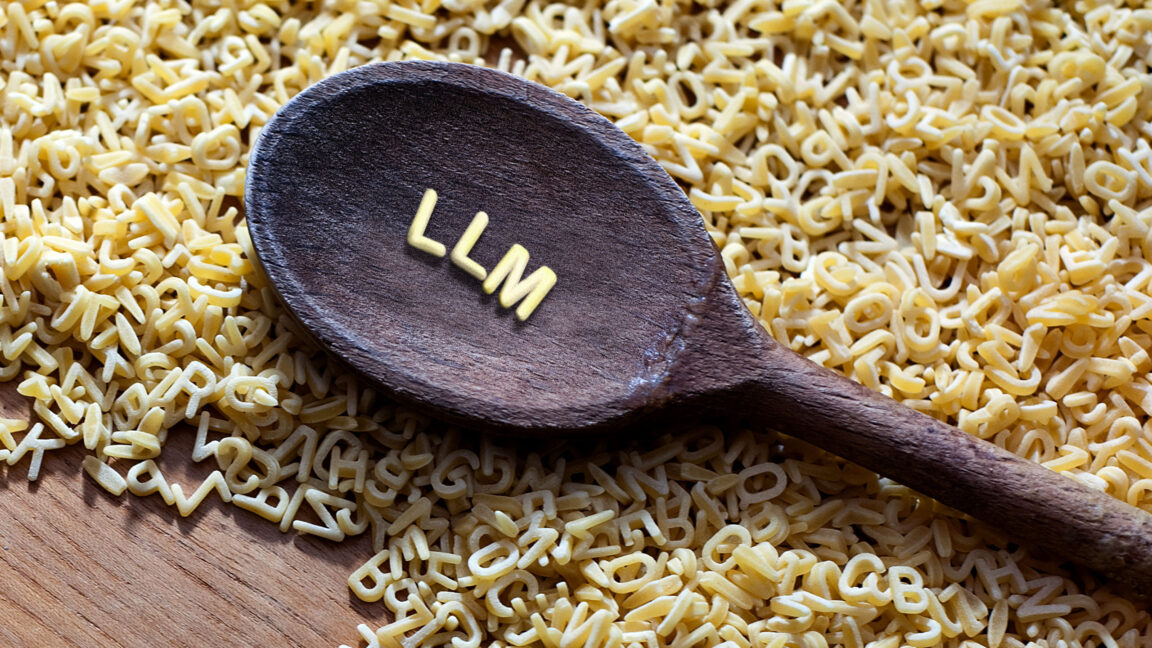
Credit: Aurich Lawson | Getty Images
Large language models represent text using tokens, each of which is a few characters. Short words are represented by a single token (like "the" or "it"), whereas larger words may be represented by several tokens (GPT-4o represents "indivisible" with "ind," "iv," and "isible").
When OpenAI released ChatGPT two years ago, it had a memory—known as a context window—of just 8,192 tokens. That works out to roughly 6,000 words of text. This meant that if you fed it more than about 15 pages of text, it would “forget” information from the beginning of its context. This limited the size and complexity of tasks ChatGPT could handle.
Today’s LLMs are far more capable:
- OpenAI’s GPT-4o can handle 128,000 tokens (about 200 pages of text).
- Anthropic’s Claude 3.5 Sonnet can accept 200,000 tokens (about 300 pages of text).
- Google’s Gemini 1.5 Pro allows 2 million tokens (about 2,000 pages of text).
Still, it’s going to take a lot more progress if we want AI systems with human-level cognitive abilities.
Many people envision a future where AI systems are able to do many—perhaps most—of the jobs performed by humans. Yet many human workers read and hear hundreds of millions of words during our working years—and we absorb even more information from sights, sounds, and smells in the world around us. To achieve human-level intelligence, AI systems will need the capacity to absorb similar quantities of information.
Right now the most popular way to build an LLM-based system to handle large amounts of information is called retrieval-augmented generation (RAG). These systems try to find documents relevant to a user’s query and then insert the most relevant documents into an LLM’s context window.
This sometimes works better than a conventional search engine, but today’s RAG systems leave a lot to be desired. They only produce good results if the system puts the most relevant documents into the LLM’s context. But the mechanism used to find those documents—often, searching in a vector database—is not very sophisticated. If the user asks a complicated or confusing question, there’s a good chance the RAG system will retrieve the wrong documents and the chatbot will return the wrong answer.
And RAG doesn’t enable an LLM to reason in more sophisticated ways over large numbers of documents:
- A lawyer might want an AI system to review and summarize hundreds of thousands of emails.
- An engineer might want an AI system to analyze thousands of hours of camera footage from a factory floor.
- A medical researcher might want an AI system to identify trends in tens of thousands of patient records.
Each of these tasks could easily require more than 2 million tokens of context. Moreover, we’re not going to want our AI systems to start with a clean slate after doing one of these jobs. We will want them to gain experience over time, just like human workers do.
Superhuman memory and stamina have long been key selling points for computers. We’re not going to want to give them up in the AI age. Yet today’s LLMs are distinctly subhuman in their ability to absorb and understand large quantities of information.
It’s true, of course, that LLMs absorb superhuman quantities of information at training time. The latest AI models have been trained on trillions of tokens—far more than any human will read or hear. But a lot of valuable information is proprietary, time-sensitive, or otherwise not available for training.
So we’re going to want AI models to read and remember far more than 2 million tokens at inference time. And that won’t be easy.
The key innovation behind transformer-based LLMs is attention, a mathematical operation that allows a model to “think about” previous tokens. (Check out our LLM explainer if you want a detailed explanation of how this works.) Before an LLM generates a new token, it performs an attention operation that compares the latest token to every previous token. This means that conventional LLMs get less and less efficient as the context grows.
Lots of people are working on ways to solve this problem—I’ll discuss some of them later in this article. But first I should explain how we ended up with such an unwieldy architecture.
GPUs made deep learning possible
The “brains” of personal computers are central processing units (CPUs). Traditionally, chipmakers made CPUs faster by increasing the frequency of the clock that acts as its heartbeat. But in the early 2000s, overheating forced chipmakers to mostly abandon this technique.
Chipmakers started making CPUs that could execute more than one instruction at a time. But they were held back by a programming paradigm that requires instructions to mostly be executed in order.
A new architecture was needed to take full advantage of Moore’s Law. Enter Nvidia.
In 1999, Nvidia started selling graphics processing units (GPUs) to speed up the rendering of three-dimensional games like Quake III Arena. The job of these PC add-on cards was to rapidly draw thousands of triangles that made up walls, weapons, monsters, and other objects in a game.
This is not a sequential programming task: triangles in different areas of the screen can be drawn in any order. So rather than having a single processor that executed instructions one at a time, Nvidia’s first GPU had a dozen specialized cores—effectively tiny CPUs—that worked in parallel to paint a scene.
Over time, Moore’s Law enabled Nvidia to make GPUs with tens, hundreds, and eventually thousands of computing cores. People started to realize that the massive parallel computing power of GPUs could be used for applications unrelated to video games.
In 2012, three University of Toronto computer scientists—Alex Krizhevsky, Ilya Sutskever, and Geoffrey Hinton—used a pair of Nvidia GTX 580 GPUs to train a neural network for recognizing images. The massive computing power of those GPUs, which had 512 cores each, allowed them to train a network with a then-impressive 60 million parameters. They entered ImageNet, an academic competition to classify images into one of 1,000 categories, and set a new record for accuracy in image recognition.
Before long, researchers were applying similar techniques to a wide variety of domains, including natural language.
Transformers removed a bottleneck for natural language
In the early 2010s, recurrent neural networks (RNNs) were a popular architecture for understanding natural language. RNNs process language one word at a time. After each word, the network updates its hidden state, a list of numbers that reflects its understanding of the sentence so far.
RNNs worked fairly well on short sentences, but they struggled with longer ones—to say nothing of paragraphs or longer passages. When reasoning about a long sentence, an RNN would sometimes “forget about” an important word early in the sentence. In 2014, computer scientists Dzmitry Bahdanau, Kyunghyun Cho, and Yoshua Bengio discovered they could improve the performance of a recurrent neural network by adding an attention mechanism that allowed the network to “look back” at earlier words in a sentence.
In 2017, Google published “Attention Is All You Need,” one of the most important papers in the history of machine learning. Building on the work of Bahdanau and his colleagues, Google researchers dispensed with the RNN and its hidden states. Instead, Google’s model used an attention mechanism to scan previous words for relevant context.
This new architecture, which Google called the transformer, proved hugely consequential because it eliminated a serious bottleneck to scaling language models.
Here’s an animation illustrating why RNNs didn’t scale well:
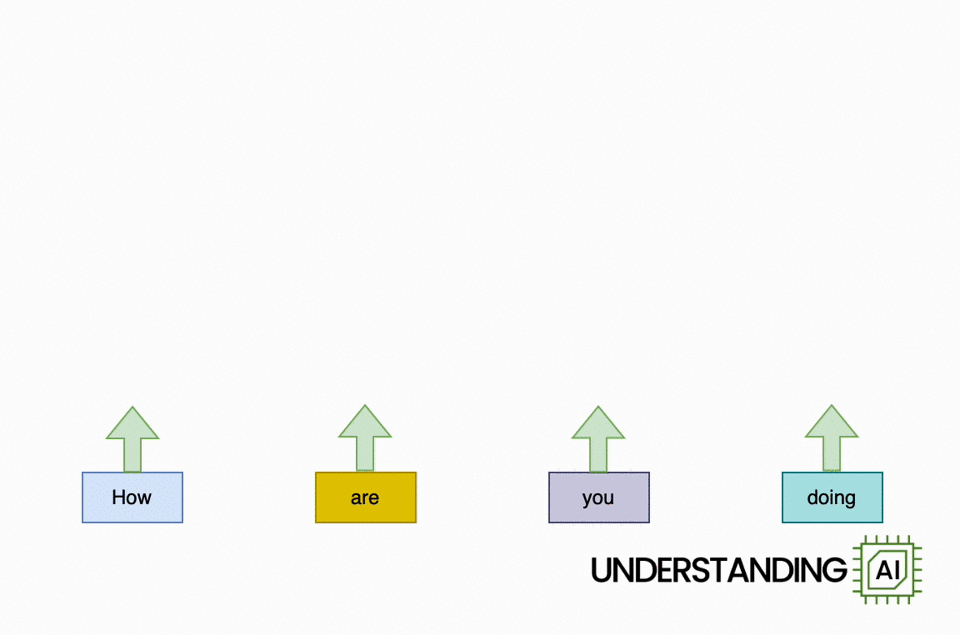
This hypothetical RNN tries to predict the next word in a sentence, with the prediction shown in the top row of the diagram. This network has three layers, each represented by a rectangle. It is inherently linear: it has to complete its analysis of the first word, “How,” before passing the hidden state back to the bottom layer so the network can start to analyze the second word, “are.”
This constraint wasn’t a big deal when machine learning algorithms ran on CPUs. But when people started leveraging the parallel computing power of GPUs, the linear architecture of RNNs became a serious obstacle.
The transformer removed this bottleneck by allowing the network to “think about” all the words in its input at the same time:
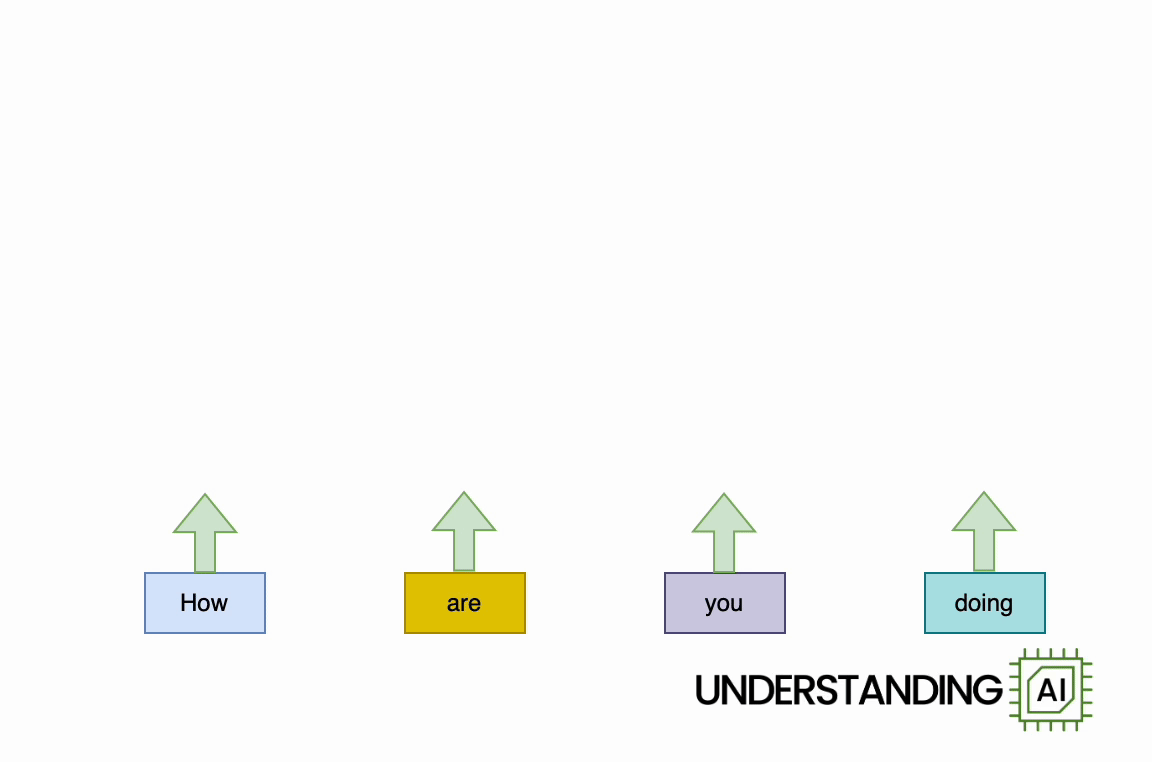