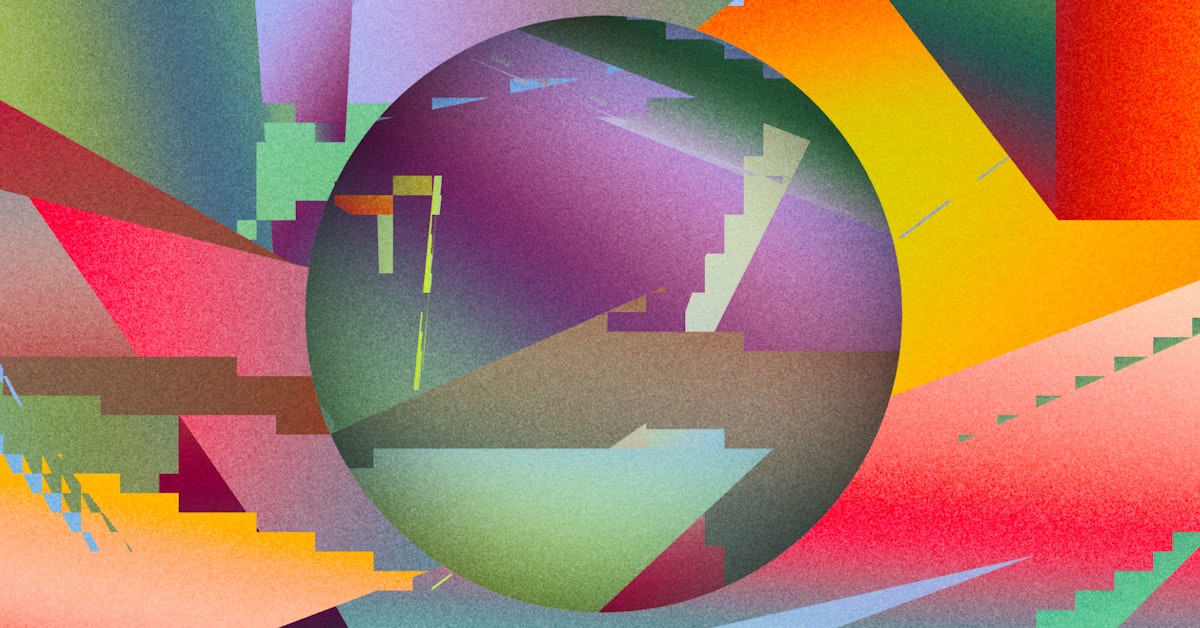
Artificial General Intelligence Is Already Here | NOEMA
Today’s most advanced AI models have many flaws, but decades from now, they will be recognized as the first true examples of artificial general intelligence.

Artificial General Intelligence Is Already Here
Today’s most advanced AI models have many flaws, but decades from now, they will be recognized as the first true examples of artificial general intelligence.Cecilia Erlich for Noema Magazine
ESSAYTECHNOLOGY & THE HUMAN
BY BLAISE AGÜERA Y ARCAS AND PETER NORVIGOCTOBER 10, 2023
Blaise Agüera y Arcas is a vice president and fellow at Google Research, where he leads an organization working on basic research, product development and infrastructure for AI.
Peter Norvig is a computer scientist and Distinguished Education Fellow at the Stanford Institute for Human-Centered AI.
Artificial General Intelligence (AGI) means many different things to different people, but the most important parts of it have already been achieved by the current generation of advanced AI large language models such as ChatGPT, Bard, LLaMA and Claude. These “frontier models” have many flaws: They hallucinate scholarly citations and court cases, perpetuate biases from their training data and make simple arithmetic mistakes. Fixing every flaw (including those often exhibited by humans) would involve building an artificial superintelligence, which is a whole other project.
Nevertheless, today’s frontier models perform competently even on novel tasks they were not trained for, crossing a threshold that previous generations of AI and supervised deep learning systems never managed. Decades from now, they will be recognized as the first true examples of AGI, just as the 1945 ENIAC is now recognized as the first true general-purpose electronic computer.
The ENIAC could be programmed with sequential, looping and conditional instructions, giving it a general-purpose applicability that its predecessors, such as the Differential Analyzer, lacked. Today’s computers far exceed ENIAC’s speed, memory, reliability and ease of use, and in the same way, tomorrow’s frontier AI will improve on today’s.
But the key property of generality? It has already been achieved.
What Is General Intelligence?
Early AI systems exhibited artificial narrow intelligence, concentrating on a single task and sometimes performing it at near or above human level. MYCIN, a program developed by Ted Shortliffe at Stanford in the 1970s, only diagnosed and recommended treatment for bacterial infections. SYSTRAN only did machine translation. IBM’s Deep Blue only played chess.Later deep neural network models trained with supervised learning such as AlexNet and AlphaGo successfully took on a number of tasks in machine perception and judgment that had long eluded earlier heuristic, rule-based or knowledge-based systems.
Most recently, we have seen frontier models that can perform a wide variety of tasks without being explicitly trained on each one. These models have achieved artificial general intelligence in five important ways:
- Topics: Frontier models are trained on hundreds of gigabytes of text from a wide variety of internet sources, covering any topic that has been written about online. Some are also trained on large and varied collections of audio, video and other media.
- Tasks: These models can perform a variety of tasks, including answering questions, generating stories, summarizing, transcribing speech, translating language, explaining, making decisions, doing customer support, calling out to other services to take actions, and combining words and images.
- Modalities: The most popular models operate on images and text, but some systems also process audio and video, and some are connected to robotic sensors and actuators. By using modality-specific tokenizers or processing raw data streams, frontier models can, in principle, handle any known sensory or motor modality.
- Languages: English is over-represented in the training data of most systems, but large models can converse in dozens of languages and translate between them, even for language pairs that have no example translations in the training data. If code is included in the training data, increasingly effective “translation” between natural languages and computer languages is even supported (i.e., general programming and reverse engineering).
- Instructability: These models are capable of “in-context learning,” where they learn from a prompt rather than from the training data. In “few-shot learning,” a new task is demonstrated with several example input/output pairs, and the system then gives outputs for novel inputs. In “zero-shot learning,” a novel task is described but no examples are given (for instance, “Write a poem about cats in the style of Hemingway” or “’Equiantonyms’ are pairs of words that are opposite of each other and have the same number of letters. What are some ‘equiantonyms’?”).
“General intelligence” must be thought of in terms of a multidimensional scorecard, not a single yes/no proposition. Nonetheless, there is a meaningful discontinuity between narrow and general intelligence: Narrowly intelligent systems typically perform a single or predetermined set of tasks, for which they are explicitly trained. Even multitask learning yields only narrow intelligence because the models still operate within the confines of tasks envisioned by the engineers. Indeed, much of the hard engineering work involved in developing narrow AI amounts to curating and labeling task-specific datasets.
By contrast, frontier language models can perform competently at pretty much any information task that can be done by humans, can be posed and answered using natural language, and has quantifiable performance.
The ability to do in-context learning is an especially meaningful meta-task for general AI. In-context learning extends the range of tasks from anything observed in the training corpus to anything that can be described, which is a big upgrade. A general AI model can perform tasks the designers never envisioned.
So: Why the reluctance to acknowledge AGI?
Frontier models have achieved a significant level of general intelligence, according to the everyday meanings of those two words. And yet most commenters have been reluctant to say so for, it seems to us, four main reasons:
- A healthy skepticism about metrics for AGI
- An ideological commitment to alternative AI theories or techniques
- A devotion to human (or biological) exceptionalism
- A concern about the economic implications of AGI
Metrics
There is a great deal of disagreement on where the threshold to AGI lies. Some people try to avoid the term altogether; Mustafa Suleyman has suggested a switch to “Artificial Capable Intelligence,” which he proposes be measured by a “modern Turing Test”: the ability to quickly make a million dollars online (from an initial $100,000 investment). AI systems able to directly generate wealth will certainly have an effect on the world, though equating “capable” with “capitalist” seems dubious.There is good reason to be skeptical of some of the metrics. When a human passes a well-constructed law, business or medical exam, we assume the human is not only competent at the specific questions on the exam, but also at a range of related questions and tasks — not to mention the broad competencies that humans possess in general. But when a frontier model is trained to pass such an exam, the training is often narrowly tuned to the exact types of questions on the test. Today’s frontier models are of course not fully qualified to be lawyers or doctors, even though they can pass those qualifying exams. As Goodhart’s law states: “When a measure becomes a target, it ceases to be a good measure.” Better tests are needed, and there is much ongoing work, such as Stanford’s test suite HELM (Holistic Evaluation of Language Models).
It is also important not to confuse linguistic fluency with intelligence. Previous generations of chatbots such as Mitsuku (now known as Kuki) could occasionally fool human judges by abruptly changing the subject and echoing a coherent passage of text. Current frontier models generate responses on the fly rather than relying on canned text, and they are better at sticking to the subject. But they still benefit from a human’s natural assumption that a fluent, grammatical response most likely comes from an intelligent entity. We call this the “Chauncey Gardiner effect,” after the hero in “Being There” — Chauncey is taken very seriously solely because he looks like someone who should be taken seriously.
The researchers Rylan Schaeffer, Brando Miranda and Sanmi Koyejo have pointed out another issue with common AI performance metrics: They are nonlinear. Consider a test consisting of a series of arithmetic problems with five-digit numbers. Small models will answer all these problems wrong, but as the size of the model is scaled up, there will be a critical threshold after which the model will get most of the problems right. This has led commenters to say that arithmetic skill is an emergent property in frontier models of sufficient size. But if instead the test included arithmetic problems with one- to four-digit numbers as well, and if partial credit were given for getting some of the digits correct, then we would see that performance increases gradually as the model size increases; there is no sharp threshold.
This finding casts doubt on the idea that super-intelligent abilities and properties, possibly including consciousness, could suddenly and mysteriously “emerge,” a fear among some citizens and policymakers. (Sometimes, the same narrative is used to “explain” why humans are intelligent while the other great apes are supposedly not; in reality, this discontinuity may be equally illusory.) Better metrics reveal that general intelligence is continuous: “More is more,” as opposed to “more is different.”
“Frontier language models can perform competently at pretty much any information task that can be done by humans, can be posed and answered using natural language, and has quantifiable performance.”