Offline Video-LLMs Can Now Understand Real-Time Streams: Apple Researchers Introduce StreamBridge to Enable Multi-Turn and Proactive Video Understanding
Offline Video-LLMs Can Now Understand Real-Time Streams: Apple Researchers Introduce StreamBridge to Enable Multi-Turn and Proactive Video Understanding
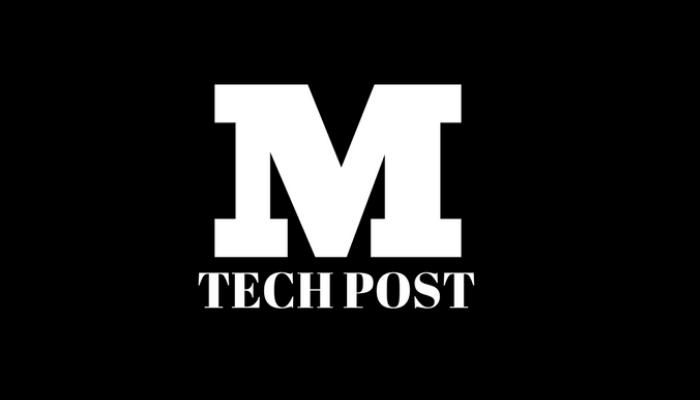
Offline Video-LLMs Can Now Understand Real-Time Streams: Apple Researchers Introduce StreamBridge to Enable Multi-Turn and Proactive Video Understanding
By Sajjad Ansari
May 12, 2025
Video-LLMs process whole pre-recorded videos at once. However, applications like robotics and autonomous driving need causal perception and interpretation of visual information online. This fundamental mismatch shows a limitation of current Video-LLMs, as they are not naturally designed to operate in streaming scenarios where timely understanding and responsiveness are paramount. The transition from offline to streaming video understanding presents two key challenges. First, multi-turn real-time understanding requires models to process the most recent video segment while maintaining historical visual and conversational context. Second, proactive response generation demands human-like behavior where the model actively monitors the visual stream and provides timely outputs based on unfolding content without explicit prompts.
Video-LLMs have gained significant attention for video understanding, combining visual encoders, modality projectors, and LLMs to generate contextual responses from video content. Several approaches have emerged to address the challenge of streaming video understanding. VideoLLMOnline and Flash-VStream introduced specialized online objectives and memory architectures for handling sequential inputs. MMDuet and ViSpeak developed dedicated components for proactive response generation. Multiple benchmark suites have been used to evaluate streaming capabilities, including StreamingBench, StreamBench, SVBench, OmniMMI, and OVO-Bench.
Researchers from Apple and Fudan University have proposed StreamBridge, a framework to transform offline Video-LLMs into streaming-capable models. It addresses two fundamental challenges in adapting existing models into online scenarios: limited capability for multi-turn real-time understanding and lack of proactive response mechanisms. StreamBridge combines a memory buffer with a round-decayed compression strategy, supporting long-context interactions. It also incorporates a decoupled, lightweight activation model that integrates seamlessly with existing Video-LLMs for proactive response generation. Further, researchers introduced Stream-IT, a large-scale dataset designed for streaming video understanding, featuring mixed videotext sequences and diverse instruction formats.
StreamBridge framework is evaluated using mainstream offline Video-LLMs, LLaVA-OV-7B, Qwen2-VL-7B, and Oryx-1.5-7B. The Stream-IT dataset is added with approximately 600K samples from established datasets to maintain general video understanding capabilities, including LLaVA-178K, VCG-Plus, and ShareGPT4Video. OVO-Bench and StreamingBench are used for multi-turn real-time understanding, focusing on their real-time tasks. General video understanding is evaluated across seven benchmarks, including three short-video datasets (MVBench, PerceptionTest, TempCompass) and four long-video benchmarks (EgoSchema, LongVideoBench, MLVU, VideoMME).
The evaluation results show that Qwen2-VL † improved with average scores increasing from 55.98 to 63.35 on OVO-Bench and 69.04 to 72.01 on Streaming-Bench. In contrast, LLaVA-OV † experiences slight performance decreases, dropping from 64.02 to 61.64 on OVO-Bench and from 71.12 to 68.39 on Streaming-Bench. Fine-tuning on the Stream-IT dataset yields substantial improvements across all models. Oryx-1.5 † achieves gains of +11.92 on OVO-Bench and +4.2 on Streaming-Bench. Moreover, Qwen2-VL † reaches average scores of 71.30 on OVO-Bench and 77.04 on Streaming-Bench after Stream-IT fine-tuning, outperforming even proprietary models like GPT-4o and Gemini 1.5 Pro, showing the effectiveness of StreamBridge’s approach in enhancing streaming video understanding capabilities.
In conclusion, researchers introduced StreamBridge, a method to transform offline Video-LLMs into effective streaming-capable models. Its dual innovations, a memory buffer with round-decayed compression strategy and a decoupled lightweight activation model, address the core challenges of streaming video understanding without compromising general performance. Further, the Stream-IT dataset is introduced for streaming video understanding, with specialized interleaved video-text sequences. As streaming video understanding becomes increasingly essential in robotics and autonomous driving, StreamBridge offers a generalizable solution that transforms static Video-LLMs into dynamic, responsive systems capable of meaningful interaction in continuously evolving visual environments.
Check out the Paper . All credit for this research goes to the researchers of this project. Also, feel free to follow us on Twitter and don’t forget to join our 90k+ ML SubReddit .
Here’s a brief overview of what we’re building at Marktechpost:
- ML News Community – r/machinelearningnews (92k+ members)
- Newsletter– airesearchinsights.com/ (30k+ subscribers)
- miniCON AI Events – minicon.marktechpost.com
- AI Reports & Magazines – magazine.marktechpost.com
- AI Dev & Research News – marktechpost.com (1M+ monthly readers)
- Partner with us