oxen.ai
GitHub
20–26 minutes
Group Relative Policy Optimization (GRPO) has proven to be a useful algorithm for training LLMs to reason and improve on benchmarks. DeepSeek-R1 showed that you can bootstrap a model through a combination of supervised fine-tuning and GRPO to compete with the state of the art models such as OpenAI's o1.
To learn more about how it works in practice, we wanted to try out some of the techniques on a real world task. This post will outline how to train your own custom small LLM using GRPO, your own data, and custom reward functions. Below is a sneak preview of some of the training curves we will see later. It is quite entertaining to watch the model learn to generate code blocks, get better at generating valid code that compiles, and finally code that passes unit tests.
If you want to jump straight into the action, the GitHub repository can be found here.
GitHub - Oxen-AI/GRPO-With-Cargo-Feedback: This repository has code for fine-tuning LLMs with GRPO specifically for Rust Programming using cargo as feedback
This repository has code for fine-tuning LLMs with GRPO specifically for Rust Programming using cargo as feedback - Oxen-AI/GRPO-With-Cargo-Feedback
Oxen-AI
This post will not go into the fundamentals of GRPO, if you want to learn more about how it works at a fundamental level, feel free to checkout our deep dive into the algorithm below.
Why GRPO is Important and How it Works | Oxen.ai
Last week on Arxiv Dives we dug into research behind DeepSeek-R1, and uncovered that one of the techniques they use in the their training pipeline is called Group Relative Policy Optimization (GRPO). At it’s core, GRPO is a Reinforcement Learning (RL) algorithm that is aimed at improving the model’s reasoning ability. It was first introduced in their paper DeepSeekMath: Pushing the Limits of Mathematical Reasoning in Open Language Models, but was also used in the post-training of DeepSeek-R1.
Oxen.ai
Rust seems like it would be a great playground for Reinforcement Learning (RL) because you have access to the rust compiler and the cargo tooling. The Rust compiler gives great error messages and is pretty strict.
In this project, the first experiment we wanted to prove out was that you can use cargo as a feedback mechanism to teach a model to become a better programmer. The second experiment we wanted to try was to see how small of a language model can you get away with. These experiments are purposely limited to a single node H100 to limit costs and show how accessible the training can be.
We are also a Rust dev shop at Oxen.ai, so have some interesting applications
x
.
Recently, there is a lot of work seeing how far we can push the boundaries of small language models for specific tasks. When you have a concrete feedback mechanism such as the correct answer to a math problem or the output of a program, it seems you can shrink the model while maintaining very competitive performance.
The rStar-Math paper from Microsoft shows this in the domain of verifiable math problems allowing the model to reason. The 1.5B model outperforms GPT-4o and o1-preview.
rStar-Math: Small LLMs Can Master Math Reasoning with Self-Evolved Deep Thinking
We present rStar-Math to demonstrate that small language models (SLMs) can rival or even surpass the math reasoning capability of OpenAI o1, without distillation from superior models. rStar-Math achieves this by exercising “deep thinking” through Monte Carlo Tree Search (MCTS), where a math policy SLM performs test-time search guided by an SLM-based process reward model. rStar-Math introduces three innovations to tackle the challenges in training the two SLMs: (1) a novel code-augmented CoT data sythesis method, which performs extensive MCTS rollouts to generate step-by-step verified reasoning trajectories used to train the policy SLM; (2) a novel process reward model training method that avoids naïve step-level score annotation, yielding a more effective process preference model (PPM); (3) a self-evolution recipe in which the policy SLM and PPM are built from scratch and iteratively evolved to improve reasoning capabilities. Through 4 rounds of self-evolution with millions of synthesized solutions for 747k math problems, rStar-Math boosts SLMs’ math reasoning to state-of-the-art levels. On the MATH benchmark, it improves Qwen2.5-Math-7B from 58.8% to 90.0% and Phi3-mini-3.8B from 41.4% to 86.4%, surpassing o1-preview by +4.5% and +0.9%. On the USA Math Olympiad (AIME), rStar-Math solves an average of 53.3% (8/15) of problems, ranking among the top 20% the brightest high school math students. Code and data will be available at https://github.com/microsoft/rStar.
arXiv.orgXinyu Guan
My hypothesis is that we can push similar level of performance on coding, since you have a similar verifiable reward: Does the code compile and does it pass unit tests?
Having small coding models have many benefits including cost, throughput, data privacy, and ability to customize to your own codebase / coding practices. Plus it's just a fun challenge.
The dream would be to eventually have this small model do all the cursor-like tasks of next tab prediction, fill in the middle, and improve it’s code in an agent loop. But let’s start simple.
There are a few different ways you could structure the problem of writing code that passes unit tests. We ended up trying a few. A seemingly straightforward option would be to have a set of verifiable unit tests that must pass given the generated code. This would give us a gold standard set of verifiable answers.
After trying out this flow we found two main problems. First, if you don’t let the model see the unit tests while writing the code, it will have no sense of the interface it is writing for. Many of the errors ended up being type or naming mismatches between the code and the unit tests while evaluating against pre-built, verified unit tests.
Second, if you allow the model to see the unit tests while its writing the code, you lose out on developer experience. Unless you are a hard core “Test Driven Developer” you probably just want to send in a prompt and not think about the function definition or unit tests yet.
Rather than trying to come up with something more clever, we ended up optimizing for simplicity. We reformulated the problem to have the model generate the code and the tests within the same response.
With single pass there is a danger of the model hacking the reward function to make the functions and unit tests trivial. For example it could just have println! and no assert statements to get everything to compile and pass. We will return to putting guardrails on for this later.
Finally we add a verbose system prompt to give the model guidance on the task.
The system prompt gives the model some context in the format and style in which we are expecting the model to answer the user queries.
Before training, we need a dataset. When starting out, we did not see many datasets targeted at Rust. Many of the LLM benchmarks are targeted at Python. So the first thing we did was convert a dataset of prompts asking Pythonic questions to a dataset of Rust prompts.
We took a random 20k prompts from the Ace-Code-87k dataset. We then used Qwen 2.5 Coder 32B Instruct to write rust code and unit tests. We ran the code and unit tests through the compiler and testing framework to filter out any triples that did not pass the unit tests. This left us with 16500 prompt,code,unit_test triples that we could train and evaluate on. The dataset was split into 15000 train, 1000 test, and 500 evaluation data points.
The final data looks like the following:
ox/Rust/cargo_test_passed_train.parquet at main
This is a dataset of rust questions and generated code created to fine tune small language models on rust.. Contribute to the ox/Rust repository by creating an account on Oxen.ai
You can follow the prompts and steps by looking at these model runs:
1) Translate to Rust: https://www.oxen.ai/ox/mbrp-playground/evaluations/ce45630c-d9e8-4fac-9b41-2d41692076b3
2) Write Rust code: https://www.oxen.ai/ox/mbrp-playground/evaluations/febc562a-9bd4-4e91-88d7-a95ee676a5ed
3) Write Rust unit tests - https://www.oxen.ai/ox/mbrp-playground/evaluations/b886ddd6-b501-4db8-8ed6-0b719d0ac595
Funny enough, for the final formulation of the GRPO training we ended up throwing away the gold standard rust code and unit tests columns. With our reinforcement learning loop we only need the prompts as input. This makes it pretty easy to collect more data in the future. We’ll dive into how the single prompt as input works in the following sections. Even though we threw away the code and unit tests for training, it was nice to know the prompts are solvable.
Training a Rust 1.5B Coder LM with Reinforcement Learning (GRPO) | Oxen.ai
GitHub
20–26 minutes
Group Relative Policy Optimization (GRPO) has proven to be a useful algorithm for training LLMs to reason and improve on benchmarks. DeepSeek-R1 showed that you can bootstrap a model through a combination of supervised fine-tuning and GRPO to compete with the state of the art models such as OpenAI's o1.
To learn more about how it works in practice, we wanted to try out some of the techniques on a real world task. This post will outline how to train your own custom small LLM using GRPO, your own data, and custom reward functions. Below is a sneak preview of some of the training curves we will see later. It is quite entertaining to watch the model learn to generate code blocks, get better at generating valid code that compiles, and finally code that passes unit tests.
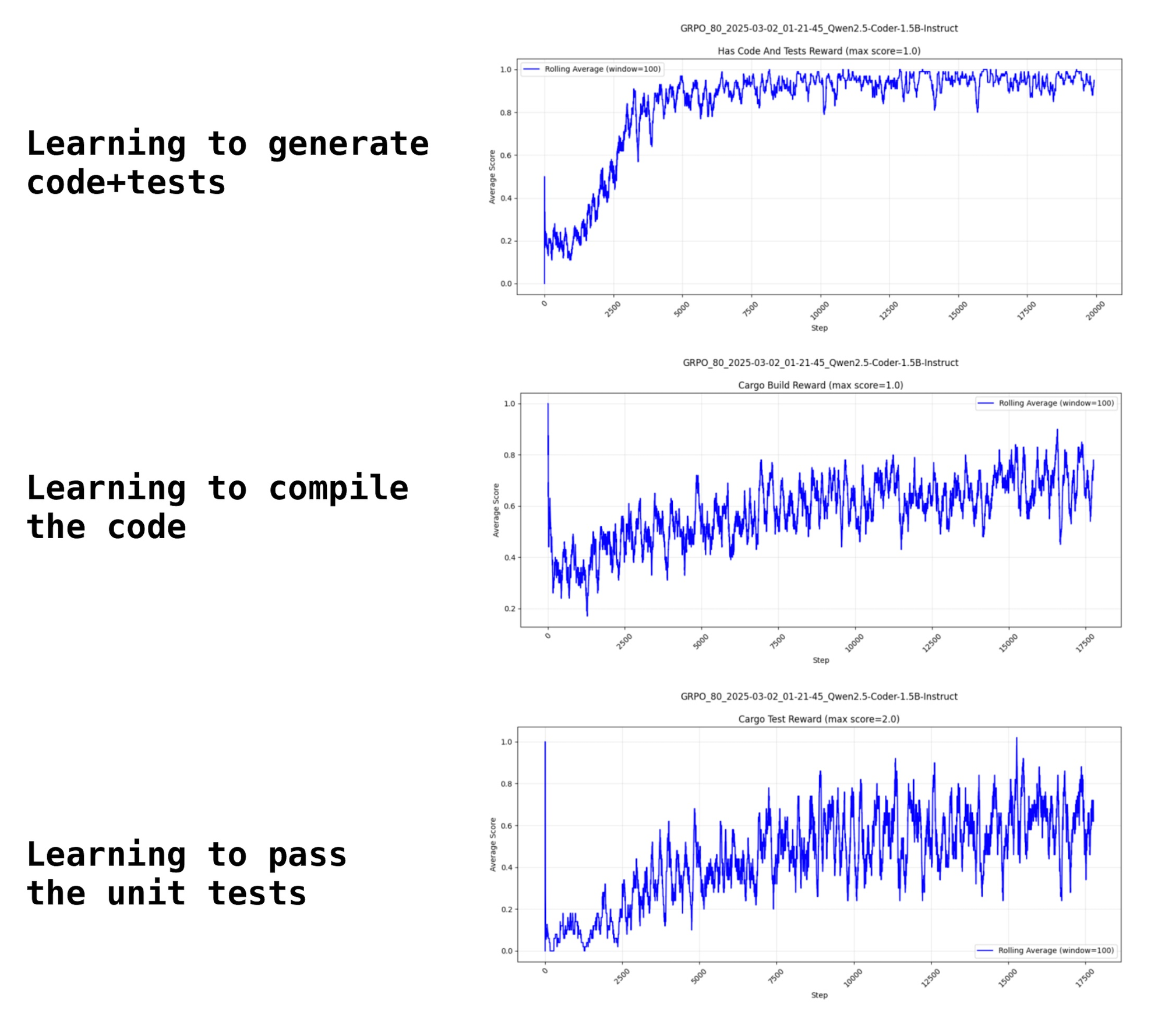
If you want to jump straight into the action, the GitHub repository can be found here.
GitHub - Oxen-AI/GRPO-With-Cargo-Feedback: This repository has code for fine-tuning LLMs with GRPO specifically for Rust Programming using cargo as feedback
This repository has code for fine-tuning LLMs with GRPO specifically for Rust Programming using cargo as feedback - Oxen-AI/GRPO-With-Cargo-Feedback
This post will not go into the fundamentals of GRPO, if you want to learn more about how it works at a fundamental level, feel free to checkout our deep dive into the algorithm below.
Why GRPO is Important and How it Works | Oxen.ai
Last week on Arxiv Dives we dug into research behind DeepSeek-R1, and uncovered that one of the techniques they use in the their training pipeline is called Group Relative Policy Optimization (GRPO). At it’s core, GRPO is a Reinforcement Learning (RL) algorithm that is aimed at improving the model’s reasoning ability. It was first introduced in their paper DeepSeekMath: Pushing the Limits of Mathematical Reasoning in Open Language Models, but was also used in the post-training of DeepSeek-R1.
Why Rust?
Rust seems like it would be a great playground for Reinforcement Learning (RL) because you have access to the rust compiler and the cargo tooling. The Rust compiler gives great error messages and is pretty strict.
In this project, the first experiment we wanted to prove out was that you can use cargo as a feedback mechanism to teach a model to become a better programmer. The second experiment we wanted to try was to see how small of a language model can you get away with. These experiments are purposely limited to a single node H100 to limit costs and show how accessible the training can be.
We are also a Rust dev shop at Oxen.ai, so have some interesting applications


Why 1.5B?
Recently, there is a lot of work seeing how far we can push the boundaries of small language models for specific tasks. When you have a concrete feedback mechanism such as the correct answer to a math problem or the output of a program, it seems you can shrink the model while maintaining very competitive performance.
The rStar-Math paper from Microsoft shows this in the domain of verifiable math problems allowing the model to reason. The 1.5B model outperforms GPT-4o and o1-preview.
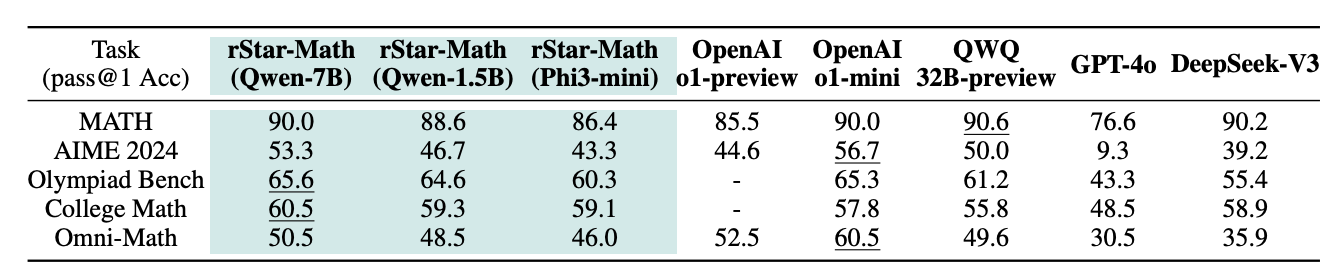
rStar-Math: Small LLMs Can Master Math Reasoning with Self-Evolved Deep Thinking
We present rStar-Math to demonstrate that small language models (SLMs) can rival or even surpass the math reasoning capability of OpenAI o1, without distillation from superior models. rStar-Math achieves this by exercising “deep thinking” through Monte Carlo Tree Search (MCTS), where a math policy SLM performs test-time search guided by an SLM-based process reward model. rStar-Math introduces three innovations to tackle the challenges in training the two SLMs: (1) a novel code-augmented CoT data sythesis method, which performs extensive MCTS rollouts to generate step-by-step verified reasoning trajectories used to train the policy SLM; (2) a novel process reward model training method that avoids naïve step-level score annotation, yielding a more effective process preference model (PPM); (3) a self-evolution recipe in which the policy SLM and PPM are built from scratch and iteratively evolved to improve reasoning capabilities. Through 4 rounds of self-evolution with millions of synthesized solutions for 747k math problems, rStar-Math boosts SLMs’ math reasoning to state-of-the-art levels. On the MATH benchmark, it improves Qwen2.5-Math-7B from 58.8% to 90.0% and Phi3-mini-3.8B from 41.4% to 86.4%, surpassing o1-preview by +4.5% and +0.9%. On the USA Math Olympiad (AIME), rStar-Math solves an average of 53.3% (8/15) of problems, ranking among the top 20% the brightest high school math students. Code and data will be available at https://github.com/microsoft/rStar.
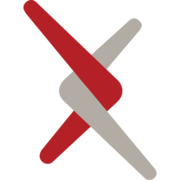
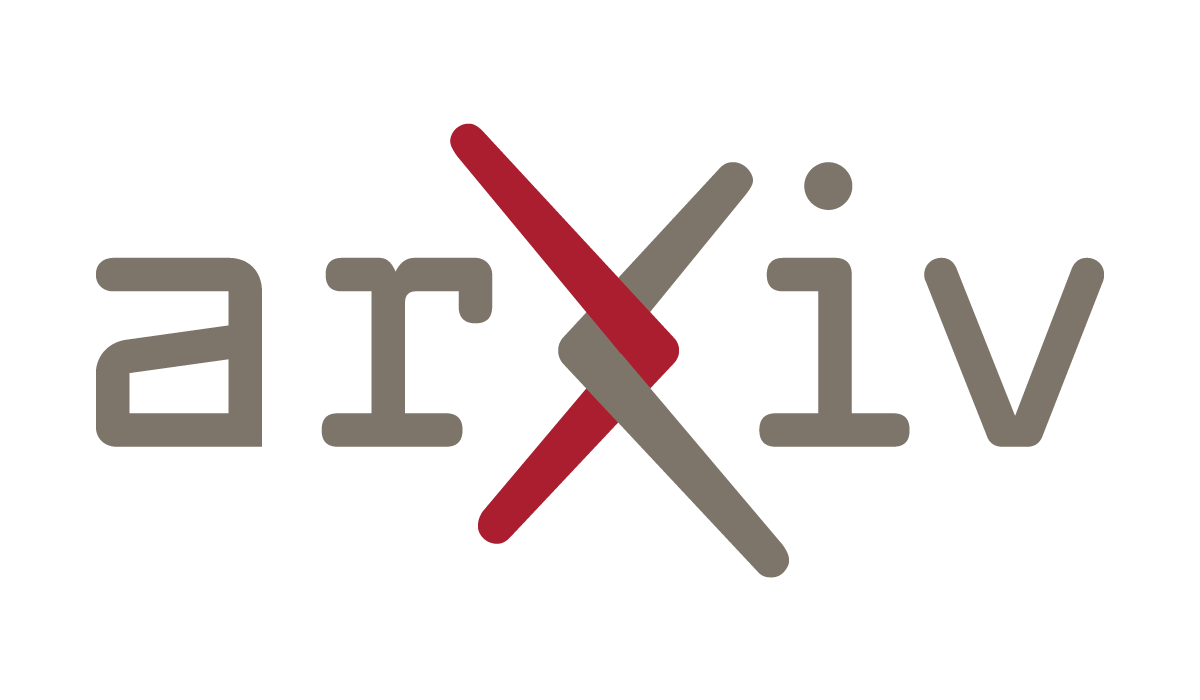
My hypothesis is that we can push similar level of performance on coding, since you have a similar verifiable reward: Does the code compile and does it pass unit tests?
Benefits of Smol LMs
Having small coding models have many benefits including cost, throughput, data privacy, and ability to customize to your own codebase / coding practices. Plus it's just a fun challenge.
The dream would be to eventually have this small model do all the cursor-like tasks of next tab prediction, fill in the middle, and improve it’s code in an agent loop. But let’s start simple.
Formulating the Problem
There are a few different ways you could structure the problem of writing code that passes unit tests. We ended up trying a few. A seemingly straightforward option would be to have a set of verifiable unit tests that must pass given the generated code. This would give us a gold standard set of verifiable answers.
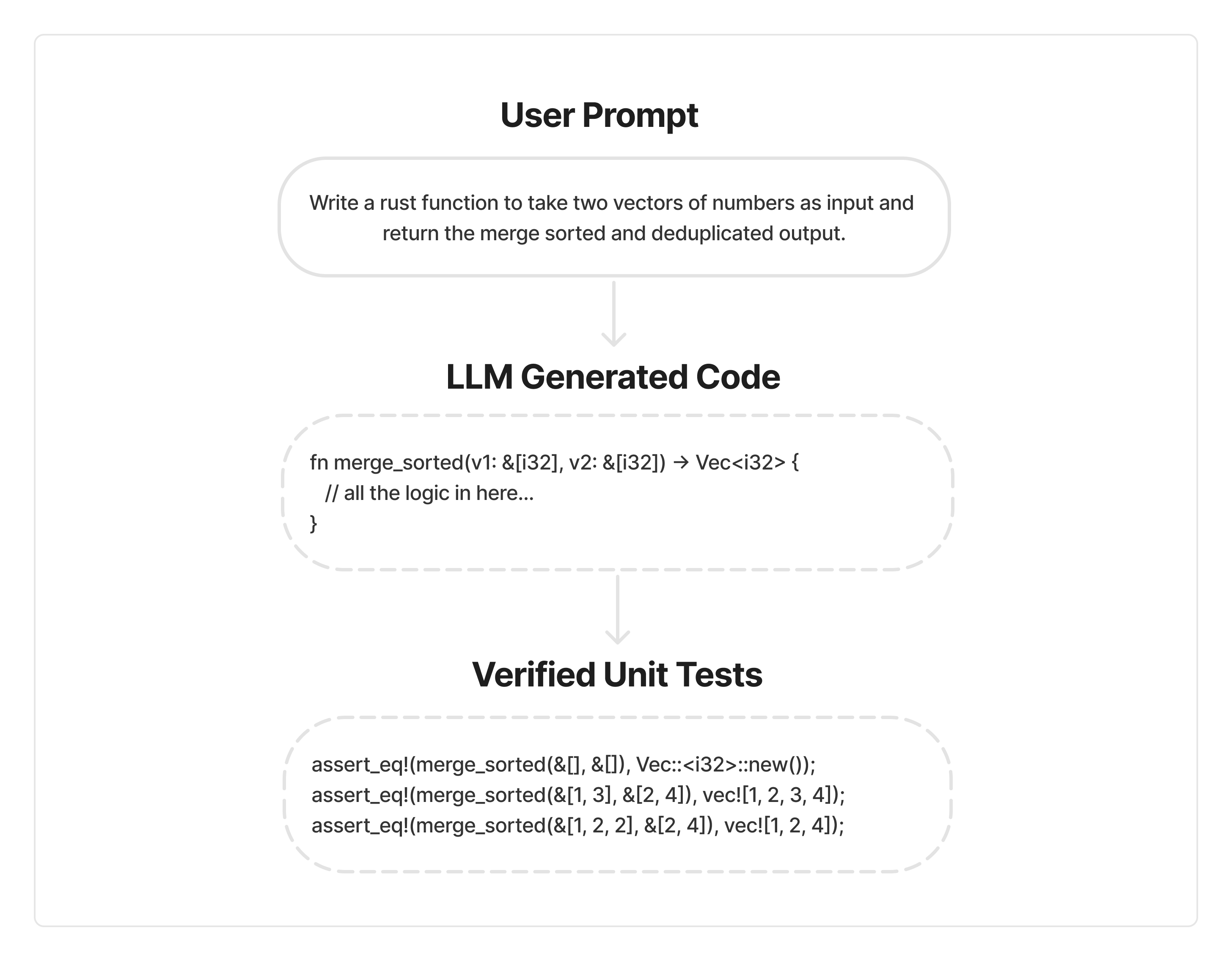
After trying out this flow we found two main problems. First, if you don’t let the model see the unit tests while writing the code, it will have no sense of the interface it is writing for. Many of the errors ended up being type or naming mismatches between the code and the unit tests while evaluating against pre-built, verified unit tests.
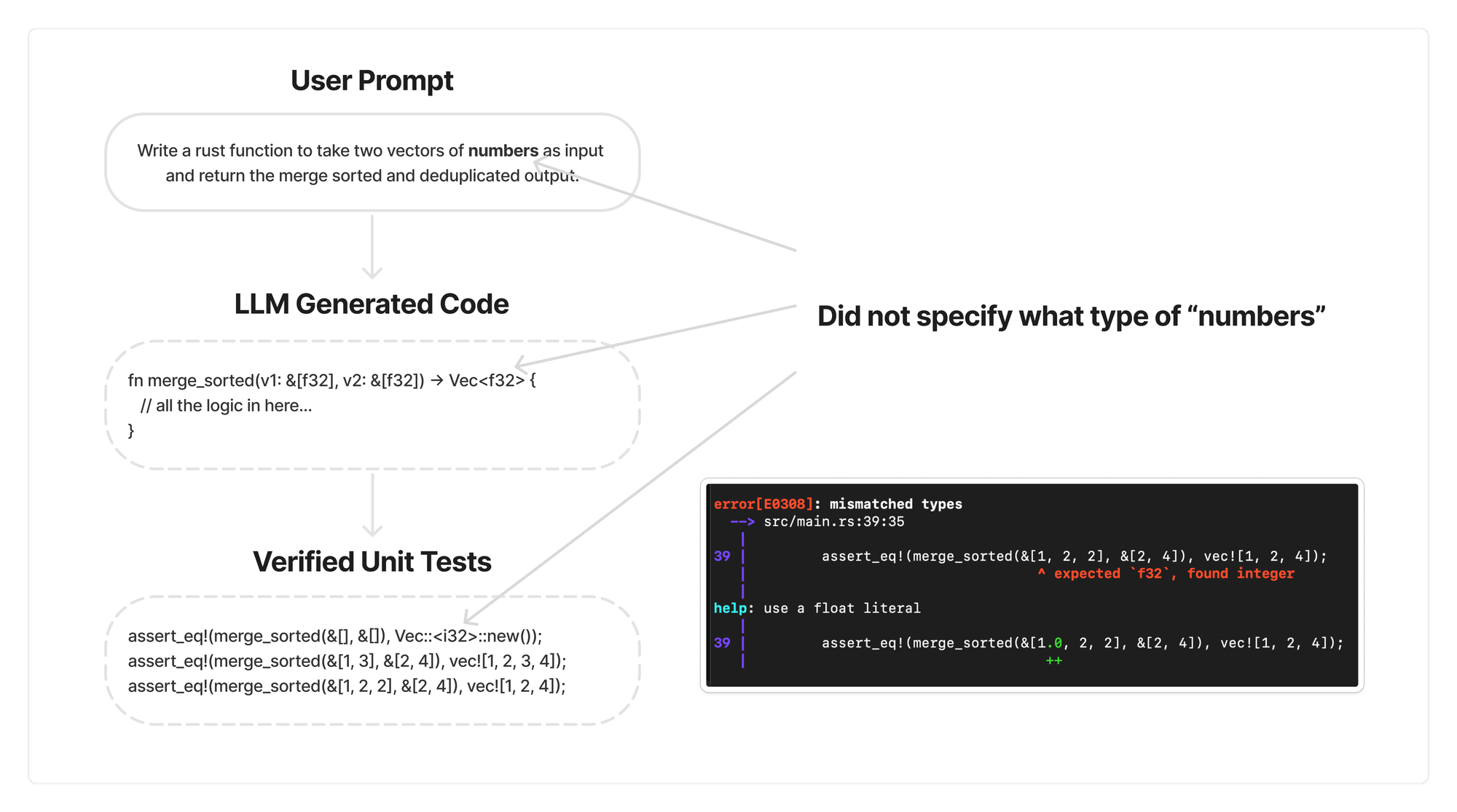
Second, if you allow the model to see the unit tests while its writing the code, you lose out on developer experience. Unless you are a hard core “Test Driven Developer” you probably just want to send in a prompt and not think about the function definition or unit tests yet.
Rather than trying to come up with something more clever, we ended up optimizing for simplicity. We reformulated the problem to have the model generate the code and the tests within the same response.
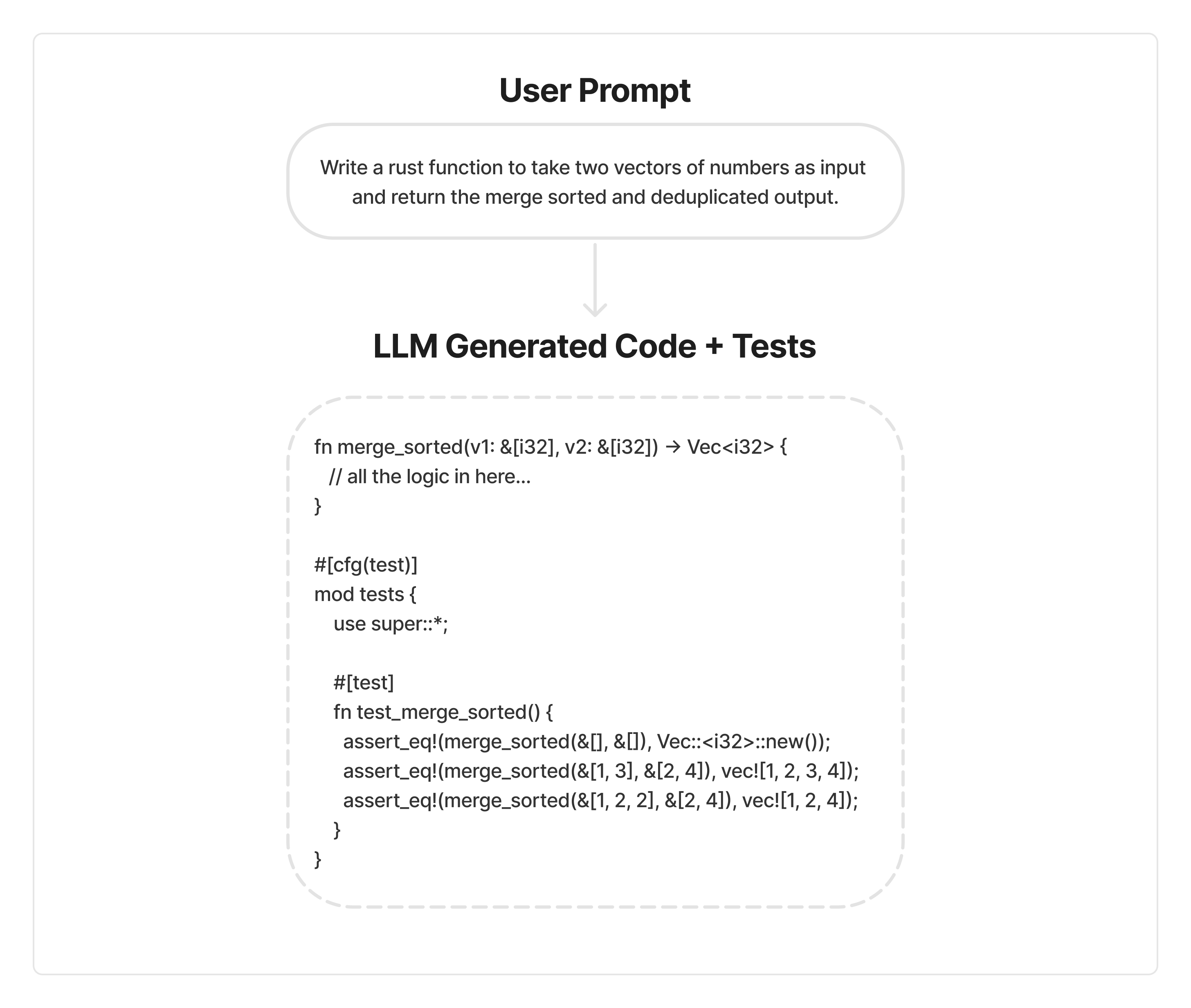
With single pass there is a danger of the model hacking the reward function to make the functions and unit tests trivial. For example it could just have println! and no assert statements to get everything to compile and pass. We will return to putting guardrails on for this later.
Finally we add a verbose system prompt to give the model guidance on the task.
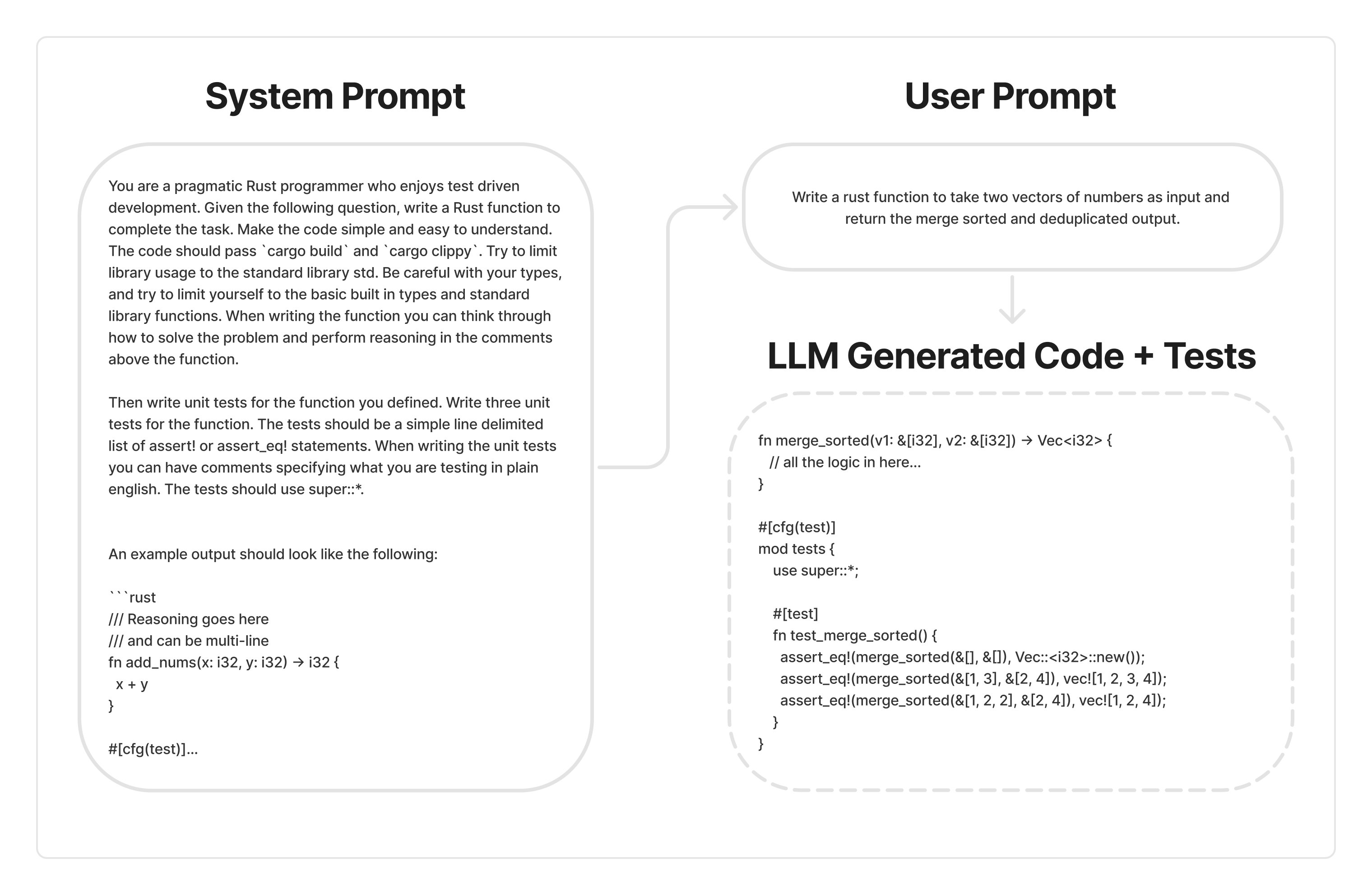
The system prompt gives the model some context in the format and style in which we are expecting the model to answer the user queries.
The Dataset
Before training, we need a dataset. When starting out, we did not see many datasets targeted at Rust. Many of the LLM benchmarks are targeted at Python. So the first thing we did was convert a dataset of prompts asking Pythonic questions to a dataset of Rust prompts.
We took a random 20k prompts from the Ace-Code-87k dataset. We then used Qwen 2.5 Coder 32B Instruct to write rust code and unit tests. We ran the code and unit tests through the compiler and testing framework to filter out any triples that did not pass the unit tests. This left us with 16500 prompt,code,unit_test triples that we could train and evaluate on. The dataset was split into 15000 train, 1000 test, and 500 evaluation data points.
The final data looks like the following:
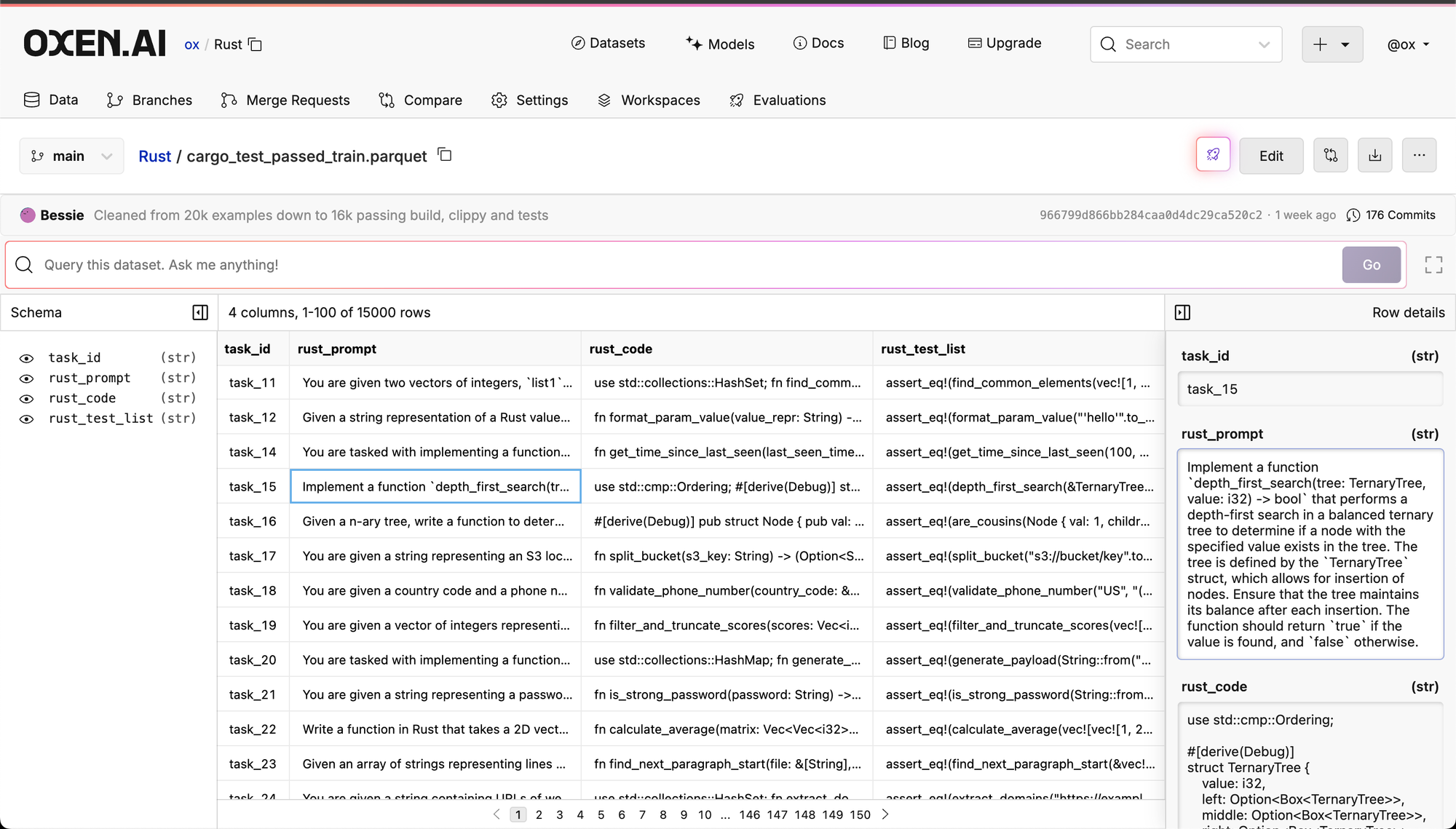
ox/Rust/cargo_test_passed_train.parquet at main
This is a dataset of rust questions and generated code created to fine tune small language models on rust.. Contribute to the ox/Rust repository by creating an account on Oxen.ai
You can follow the prompts and steps by looking at these model runs:
1) Translate to Rust: https://www.oxen.ai/ox/mbrp-playground/evaluations/ce45630c-d9e8-4fac-9b41-2d41692076b3
2) Write Rust code: https://www.oxen.ai/ox/mbrp-playground/evaluations/febc562a-9bd4-4e91-88d7-a95ee676a5ed
3) Write Rust unit tests - https://www.oxen.ai/ox/mbrp-playground/evaluations/b886ddd6-b501-4db8-8ed6-0b719d0ac595
Funny enough, for the final formulation of the GRPO training we ended up throwing away the gold standard rust code and unit tests columns. With our reinforcement learning loop we only need the prompts as input. This makes it pretty easy to collect more data in the future. We’ll dive into how the single prompt as input works in the following sections. Even though we threw away the code and unit tests for training, it was nice to know the prompts are solvable.