1/19
@ChrisClickUp
The $3B feature Google couldn't stop:
OpenAI's record-breaking acquisition wasn't for an AI assistant:
They found a way to modify specific lines of code without full codebase access.
Here's why this threatens every company's software security:
2/19
@ChrisClickUp
Most code edits today require full codebase access.
You need to see the entire program to make targeted changes.
Think of it like needing to examine an entire car just to replace a faulty headlight.
But what if you could fix that headlight without seeing the rest of the car?
https://video.twimg.com/amplify_video/1920160422625488898/vid/avc1/1280x720/jtKJdydy-6dlE0yV.mp4
3/19
@ChrisClickUp
That's what OpenAI acquired with their $3B purchase of Windsurf (formerly Codeium).
Their tech lets AI modify specific code sections without seeing the entire codebase.
This changes everything about software security...
4/19
@ChrisClickUp
Imagine a locksmith who can replace just one tumbler in your lock without taking the whole thing apart.
Sounds convenient, right?
But what if that locksmith is a thief?
That's the double-edged sword of this technology:
5/19
@ChrisClickUp
The tech works by creating a "mental model" of how code functions.
It allows users to modify individual lines through natural language prompts.
No need to download or review the entire codebase.
https://video.twimg.com/amplify_video/1920160525029421056/vid/avc1/1280x720/dZeRjlmtmPgSFSWL.mp4
6/19
@ChrisClickUp
Traditional security models rely on "security through obscurity."
Companies keep their codebases private, assuming hackers need to see the whole system to exploit it.
That assumption just collapsed.
With Windsurf's technology, attackers could potentially launch attacks with minimal code exposure.
7/19
@ChrisClickUp
Why did OpenAI pay $3 billion – more than double Windsurf's previous $1.25 billion valuation?
Because it fundamentally changes software development:
• Faster code fixes without complex system understanding
• More accessible programming for non-experts
• Automated updates across massive codebases
But these benefits come with serious security risks:
https://video.twimg.com/amplify_video/1920160573872099328/vid/avc1/1280x720/l4tyQIOukpcnnaD8.mp4
8/19
@ChrisClickUp
Every software vulnerability becomes more dangerous.
Previously, hackers finding a security flaw still needed to understand the surrounding code to exploit it.
Now, AI could potentially generate working exploits from minimal information.
This creates powerful advantages for malicious actors.
https://video.twimg.com/amplify_video/1920160621590712321/vid/avc1/1280x720/cecxG1HQ3ytPBRXA.mp4
9/19
@ChrisClickUp
• Small code leaks become major vulnerabilities
• Legacy systems become easier to compromise
• Supply chain attacks grow more sophisticated
• Open-source contributions could hide malicious code
Google reportedly tried to acquire similar technologies but couldn't match OpenAI's move.
Why were they so desperate?
https://video.twimg.com/amplify_video/1920160664288702465/vid/avc1/1280x720/-QsvBItwtr2AV3sR.mp4
10/19
@ChrisClickUp
Because whoever controls this capability shapes the future of code security.
Before being acquired, Windsurf offered free usage for developers who supplied their API keys.
The company had also partnered with several Fortune 500 firms under strict NDAs.
The security implications are enormous:
https://video.twimg.com/amplify_video/1920160696157024256/vid/avc1/1280x720/6UxQaX2rkYVPodC9.mp4
11/19
@ChrisClickUp
According to industry analysts, Windsurf's technology represents one of the most significant shifts in software security in years.
Companies must now assume that small code snippets could compromise their entire systems.
https://video.twimg.com/amplify_video/1920160762116571136/vid/avc1/1280x720/Z7qPhEnM6XJtktFu.mp4
12/19
@ChrisClickUp
Traditional security practices like:
• Code obfuscation
• Limiting repository access
• Segmenting codebases
Are no longer sufficient protections.
What should companies do instead?
13/19
@ChrisClickUp
The most effective defense will be comprehensive runtime monitoring and behavioral analysis.
Since preventing code access becomes less effective, detecting unusual behavior becomes essential.
Companies should implement:
• Simulation environments for testing changes
• Automated static analysis
• Zero-trust architecture
https://video.twimg.com/amplify_video/1920160809621336066/vid/avc1/1280x720/AfUv6WIuh8HrRgTY.mp4
14/19
@ChrisClickUp
This acquisition signals the beginning of a new arms race between AI-powered development and AI-powered security.
Companies that adapt quickly will thrive.
Those that cling to outdated security models will find themselves increasingly vulnerable.
The era of "security through obscurity" is officially over.
15/19
@ChrisClickUp
As AI continues to revolutionize code creation and modification, we're seeing a fundamental shift in how companies must approach security.
The old playbook of protecting your codebase is becoming obsolete.
What matters now is understanding how your code behaves when it runs - and identifying anomalies before they become breaches.
16/19
@ChrisClickUp
This shift requires a new generation of security tools designed specifically for the AI era.
Tools that can monitor behavior patterns in real-time.
Tools that can detect subtle code modifications that traditional security measures would miss.
Tools built by people who understand both AI and security at a fundamental level.
https://video.twimg.com/amplify_video/1920160883822804992/vid/avc1/1280x720/ARxoaJojQjvjfutd.mp4
17/19
@ChrisClickUp
That's why I've been obsessively tracking these developments in AI security for years.
Each breakthrough - from automated coding to this new surgical code modification capability - creates both possibilities and vulnerabilities.
By understanding where this technology is headed, we can build better protections and smarter systems.
18/19
@ChrisClickUp
Want to stay ahead of these emerging AI security threats and opportunities?
Follow me for weekly insights on AI developments that impact your business security.
I share practical strategies to protect your systems in this rapidly evolving landscape.
19/19
@ChrisClickUp
Video credits:
Deirdre Bosa - CNBC:
Y Combinator:
AI LABS:
Low Level:
TED:
To post tweets in this format, more info here: https://www.thecoli.com/threads/tips-and-tricks-for-posting-the-coli-megathread.984734/post-52211196
@ChrisClickUp
The $3B feature Google couldn't stop:
OpenAI's record-breaking acquisition wasn't for an AI assistant:
They found a way to modify specific lines of code without full codebase access.
Here's why this threatens every company's software security:
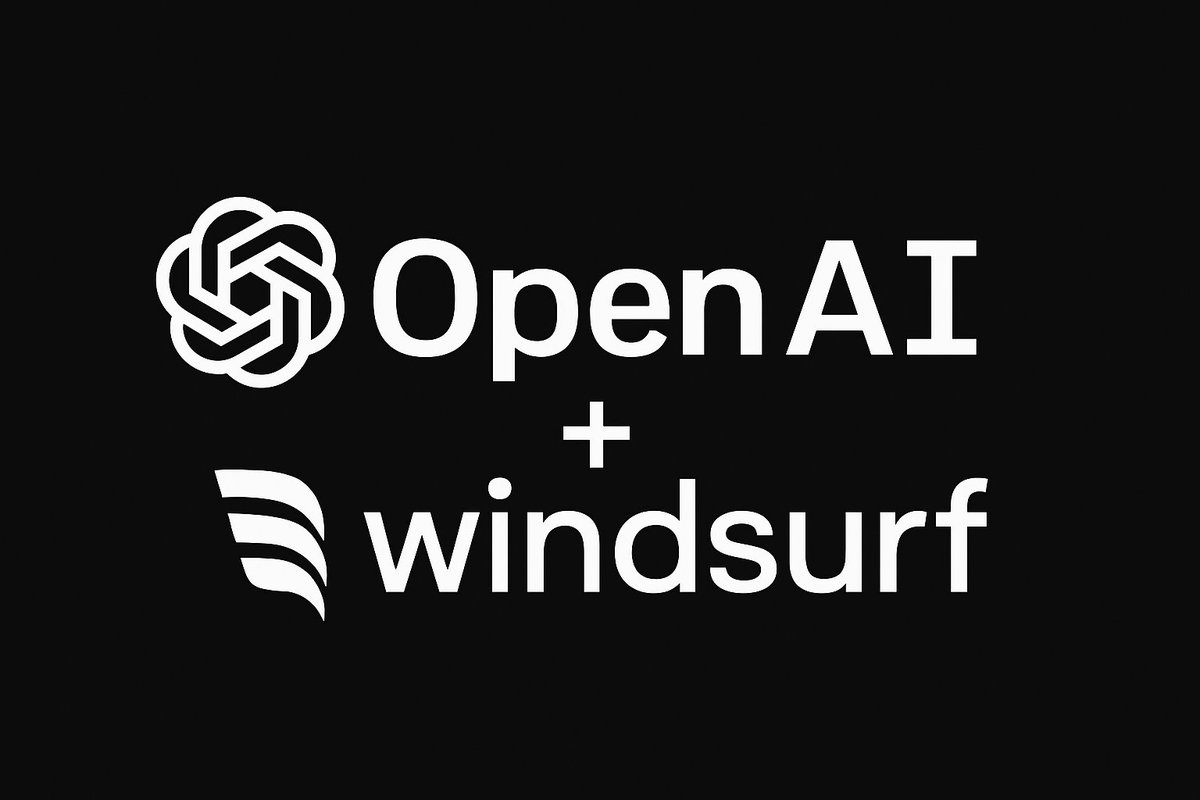
2/19
@ChrisClickUp
Most code edits today require full codebase access.
You need to see the entire program to make targeted changes.
Think of it like needing to examine an entire car just to replace a faulty headlight.
But what if you could fix that headlight without seeing the rest of the car?
https://video.twimg.com/amplify_video/1920160422625488898/vid/avc1/1280x720/jtKJdydy-6dlE0yV.mp4
3/19
@ChrisClickUp
That's what OpenAI acquired with their $3B purchase of Windsurf (formerly Codeium).
Their tech lets AI modify specific code sections without seeing the entire codebase.
This changes everything about software security...
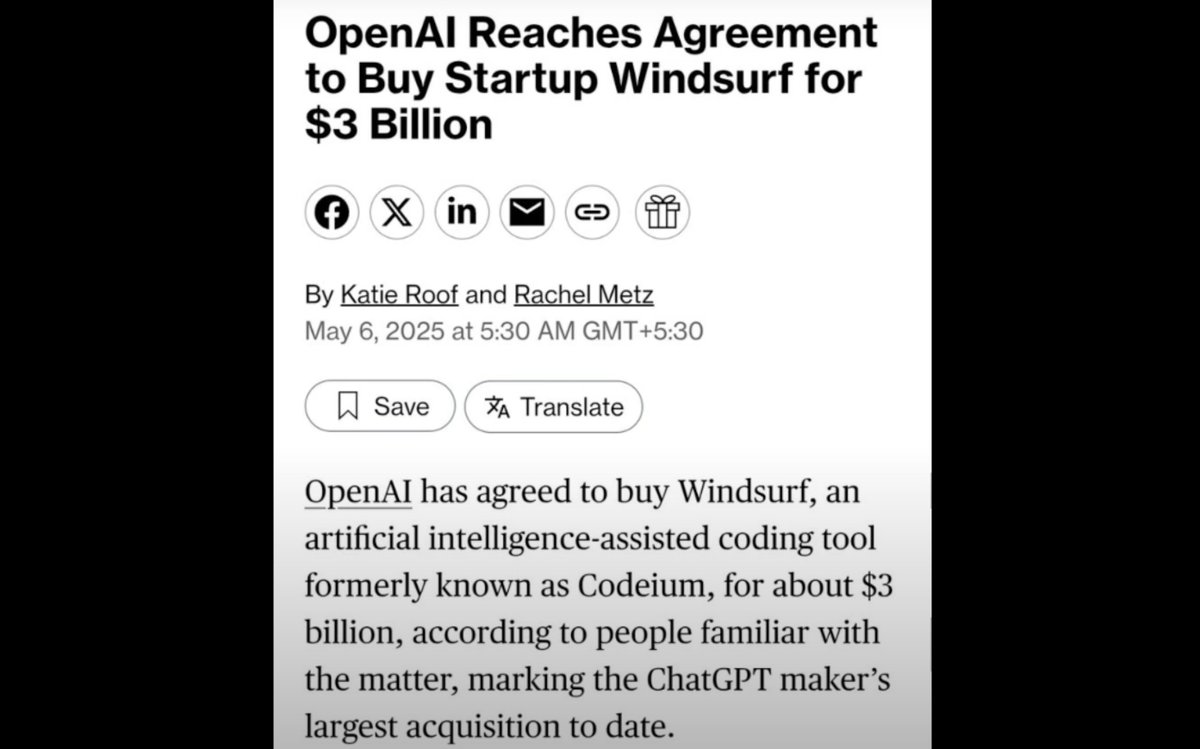
4/19
@ChrisClickUp
Imagine a locksmith who can replace just one tumbler in your lock without taking the whole thing apart.
Sounds convenient, right?
But what if that locksmith is a thief?
That's the double-edged sword of this technology:
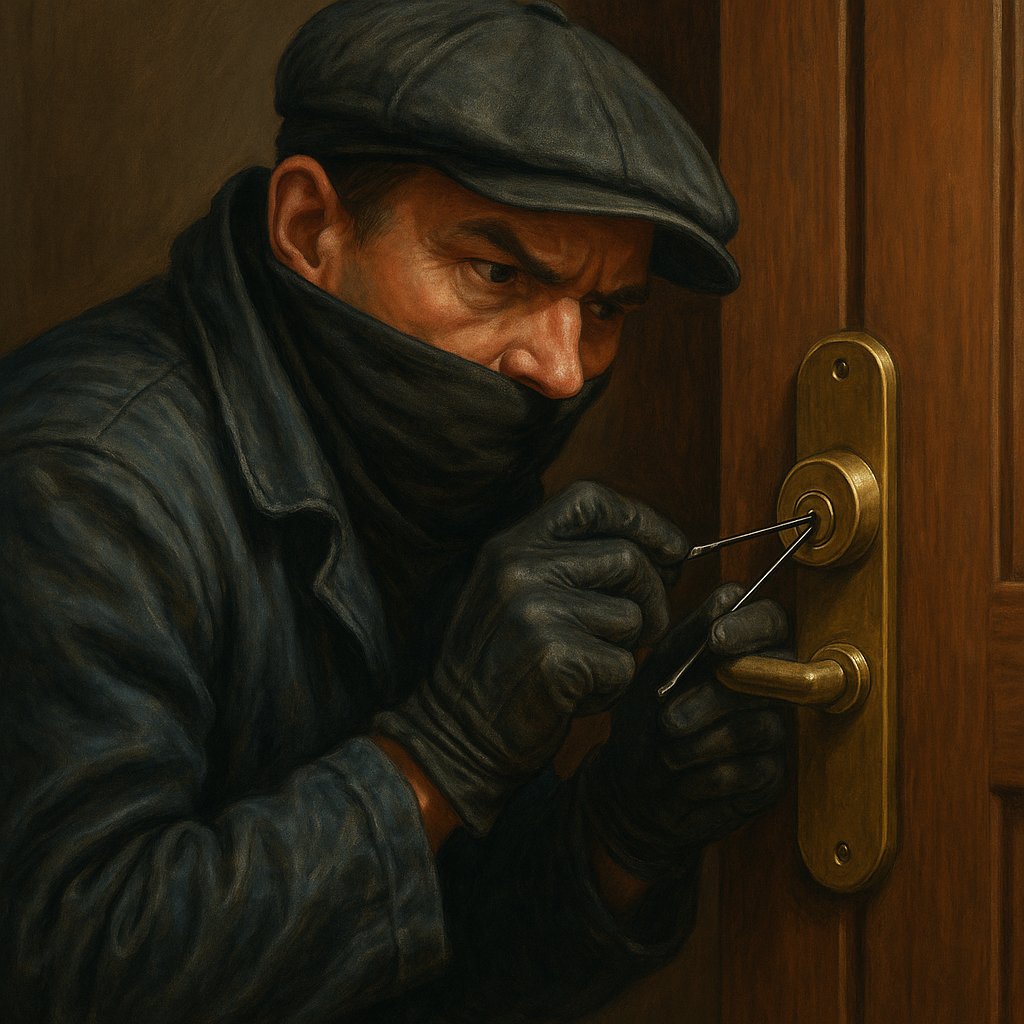
5/19
@ChrisClickUp
The tech works by creating a "mental model" of how code functions.
It allows users to modify individual lines through natural language prompts.
No need to download or review the entire codebase.
https://video.twimg.com/amplify_video/1920160525029421056/vid/avc1/1280x720/dZeRjlmtmPgSFSWL.mp4
6/19
@ChrisClickUp
Traditional security models rely on "security through obscurity."
Companies keep their codebases private, assuming hackers need to see the whole system to exploit it.
That assumption just collapsed.
With Windsurf's technology, attackers could potentially launch attacks with minimal code exposure.
7/19
@ChrisClickUp
Why did OpenAI pay $3 billion – more than double Windsurf's previous $1.25 billion valuation?
Because it fundamentally changes software development:
• Faster code fixes without complex system understanding
• More accessible programming for non-experts
• Automated updates across massive codebases
But these benefits come with serious security risks:
https://video.twimg.com/amplify_video/1920160573872099328/vid/avc1/1280x720/l4tyQIOukpcnnaD8.mp4
8/19
@ChrisClickUp
Every software vulnerability becomes more dangerous.
Previously, hackers finding a security flaw still needed to understand the surrounding code to exploit it.
Now, AI could potentially generate working exploits from minimal information.
This creates powerful advantages for malicious actors.
https://video.twimg.com/amplify_video/1920160621590712321/vid/avc1/1280x720/cecxG1HQ3ytPBRXA.mp4
9/19
@ChrisClickUp
• Small code leaks become major vulnerabilities
• Legacy systems become easier to compromise
• Supply chain attacks grow more sophisticated
• Open-source contributions could hide malicious code
Google reportedly tried to acquire similar technologies but couldn't match OpenAI's move.
Why were they so desperate?
https://video.twimg.com/amplify_video/1920160664288702465/vid/avc1/1280x720/-QsvBItwtr2AV3sR.mp4
10/19
@ChrisClickUp
Because whoever controls this capability shapes the future of code security.
Before being acquired, Windsurf offered free usage for developers who supplied their API keys.
The company had also partnered with several Fortune 500 firms under strict NDAs.
The security implications are enormous:
https://video.twimg.com/amplify_video/1920160696157024256/vid/avc1/1280x720/6UxQaX2rkYVPodC9.mp4
11/19
@ChrisClickUp
According to industry analysts, Windsurf's technology represents one of the most significant shifts in software security in years.
Companies must now assume that small code snippets could compromise their entire systems.
https://video.twimg.com/amplify_video/1920160762116571136/vid/avc1/1280x720/Z7qPhEnM6XJtktFu.mp4
12/19
@ChrisClickUp
Traditional security practices like:
• Code obfuscation
• Limiting repository access
• Segmenting codebases
Are no longer sufficient protections.
What should companies do instead?
13/19
@ChrisClickUp
The most effective defense will be comprehensive runtime monitoring and behavioral analysis.
Since preventing code access becomes less effective, detecting unusual behavior becomes essential.
Companies should implement:
• Simulation environments for testing changes
• Automated static analysis
• Zero-trust architecture
https://video.twimg.com/amplify_video/1920160809621336066/vid/avc1/1280x720/AfUv6WIuh8HrRgTY.mp4
14/19
@ChrisClickUp
This acquisition signals the beginning of a new arms race between AI-powered development and AI-powered security.
Companies that adapt quickly will thrive.
Those that cling to outdated security models will find themselves increasingly vulnerable.
The era of "security through obscurity" is officially over.
15/19
@ChrisClickUp
As AI continues to revolutionize code creation and modification, we're seeing a fundamental shift in how companies must approach security.
The old playbook of protecting your codebase is becoming obsolete.
What matters now is understanding how your code behaves when it runs - and identifying anomalies before they become breaches.
16/19
@ChrisClickUp
This shift requires a new generation of security tools designed specifically for the AI era.
Tools that can monitor behavior patterns in real-time.
Tools that can detect subtle code modifications that traditional security measures would miss.
Tools built by people who understand both AI and security at a fundamental level.
https://video.twimg.com/amplify_video/1920160883822804992/vid/avc1/1280x720/ARxoaJojQjvjfutd.mp4
17/19
@ChrisClickUp
That's why I've been obsessively tracking these developments in AI security for years.
Each breakthrough - from automated coding to this new surgical code modification capability - creates both possibilities and vulnerabilities.
By understanding where this technology is headed, we can build better protections and smarter systems.
18/19
@ChrisClickUp
Want to stay ahead of these emerging AI security threats and opportunities?
Follow me for weekly insights on AI developments that impact your business security.
I share practical strategies to protect your systems in this rapidly evolving landscape.
19/19
@ChrisClickUp
Video credits:
Deirdre Bosa - CNBC:
Y Combinator:
AI LABS:
Low Level:
TED:
To post tweets in this format, more info here: https://www.thecoli.com/threads/tips-and-tricks-for-posting-the-coli-megathread.984734/post-52211196
1/6
@kevinhou22
goodbye runbooks, hello /workflows
[1/5] absolutely LOVING this new feature in windsurf. Some of my use cases so far:
- deploy my server to kubernetes
- generate PRs using my team's style
- get the recent error logs
The possibilities are endless. Here's how it works
2/6
@kevinhou22
[2/5] Windsurf rules already provide LLMs with guidance via persistent, reusable context at the prompt level.
Workflows extend this concept with:
- structured sequences of prompts on a per step level
- chaining interconnected tasks / actions
- general enough to handle ambiguity
3/6
@kevinhou22
[3/5] It's super simple to setup a new /workflow:
1. Click "Customize" --> "Workflows"
2. Press "+Workflow" to create a new one
3. Add a series of steps that Windsurf can follow
4. Set a title & description
The best part is, you can write it all in text!
https://video.twimg.com/amplify_video/1920197094142521344/vid/avc1/3652x2160/a14EBqmsj3lhtuei.mp4
4/6
@kevinhou22
[4/5] You can also ask Windsurf to generate Workflows for you! This works particularly well for workflows involving a series of steps in a particular CLI tool.
Check it out:
https://video.twimg.com/amplify_video/1920197704044720129/vid/avc1/3696x2160/AyMiwDXJ28XE1Xy0.mp4
5/6
@kevinhou22
[5/5] To execute a workflow, users simply invoke it in Cascade using the /[workflow-name] command.
It's that easy
Check it out on @windsurf_ai v1.8.2 today
Windsurf (formerly Codeium) - The most powerful AI Code Editor
6/6
@lyson_ober
Respect 🫡 Like it
To post tweets in this format, more info here: https://www.thecoli.com/threads/tips-and-tricks-for-posting-the-coli-megathread.984734/post-52211196
@kevinhou22
goodbye runbooks, hello /workflows

[1/5] absolutely LOVING this new feature in windsurf. Some of my use cases so far:
- deploy my server to kubernetes
- generate PRs using my team's style
- get the recent error logs
The possibilities are endless. Here's how it works

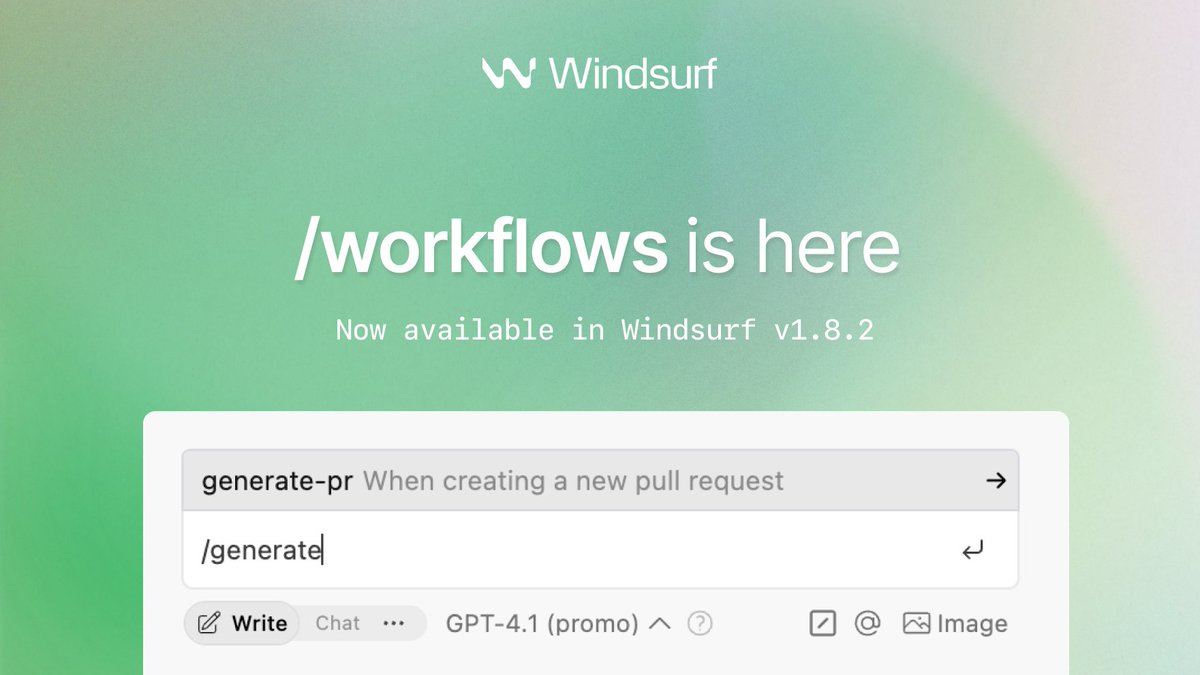
2/6
@kevinhou22
[2/5] Windsurf rules already provide LLMs with guidance via persistent, reusable context at the prompt level.
Workflows extend this concept with:
- structured sequences of prompts on a per step level
- chaining interconnected tasks / actions
- general enough to handle ambiguity
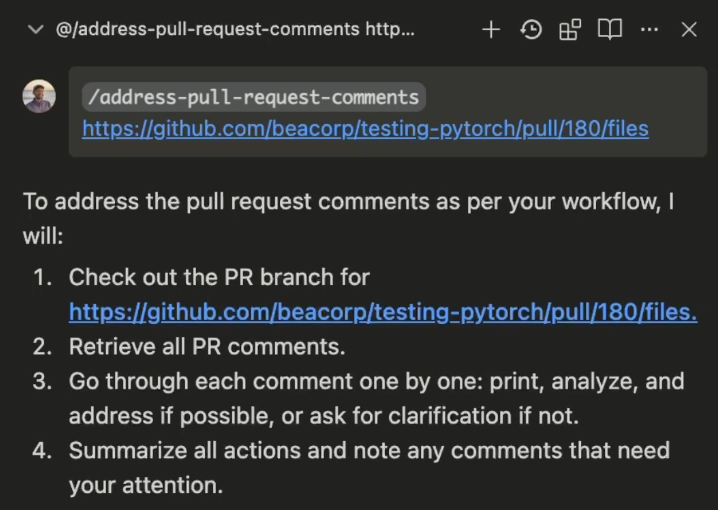
3/6
@kevinhou22
[3/5] It's super simple to setup a new /workflow:
1. Click "Customize" --> "Workflows"
2. Press "+Workflow" to create a new one
3. Add a series of steps that Windsurf can follow
4. Set a title & description
The best part is, you can write it all in text!
https://video.twimg.com/amplify_video/1920197094142521344/vid/avc1/3652x2160/a14EBqmsj3lhtuei.mp4
4/6
@kevinhou22
[4/5] You can also ask Windsurf to generate Workflows for you! This works particularly well for workflows involving a series of steps in a particular CLI tool.
Check it out:
https://video.twimg.com/amplify_video/1920197704044720129/vid/avc1/3696x2160/AyMiwDXJ28XE1Xy0.mp4
5/6
@kevinhou22
[5/5] To execute a workflow, users simply invoke it in Cascade using the /[workflow-name] command.
It's that easy
Check it out on @windsurf_ai v1.8.2 today

Windsurf (formerly Codeium) - The most powerful AI Code Editor
6/6
@lyson_ober
Respect 🫡 Like it

To post tweets in this format, more info here: https://www.thecoli.com/threads/tips-and-tricks-for-posting-the-coli-megathread.984734/post-52211196